Legal AI: Exploring Bias And Inaccuracy
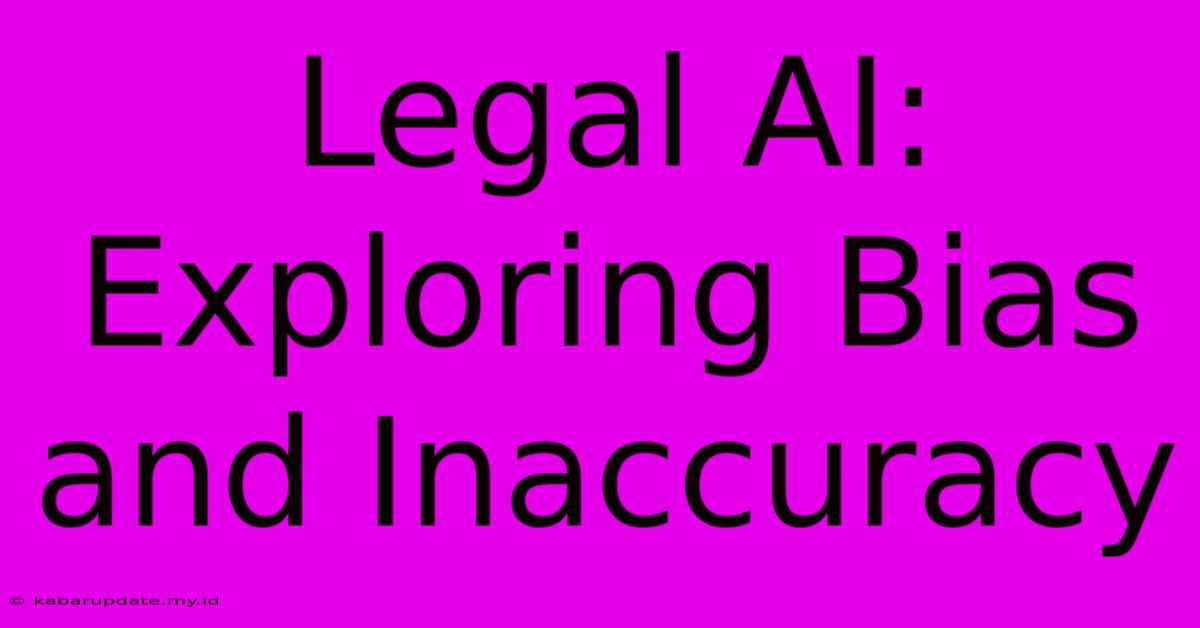
Discover more in-depth information on our site. Click the link below to dive deeper: Visit the Best Website meltwatermedia.ca. Make sure you donβt miss it!
Table of Contents
Legal AI: Exploring Bias and Inaccuracy
The rise of Artificial Intelligence (AI) is transforming numerous sectors, and the legal field is no exception. Legal AI tools promise increased efficiency, improved accuracy, and reduced costs. However, the integration of AI in legal practices also raises significant concerns regarding bias and inaccuracy. This article delves into these crucial aspects, examining their potential impact and offering strategies for mitigation.
The Perils of Bias in Legal AI
AI algorithms are trained on vast datasets of legal information. If this data reflects existing societal biases β for instance, racial, gender, or socioeconomic biases β the AI system will likely perpetuate and even amplify these biases in its outputs. This can lead to unfair or discriminatory outcomes in various legal applications.
Examples of Bias in Action:
- Predictive Policing: AI systems used to predict crime rates might disproportionately target certain demographics due to biased historical crime data, leading to unfair policing practices.
- Sentencing Recommendations: AI tools suggesting sentencing lengths could reflect existing biases in judicial decisions, potentially resulting in harsher sentences for specific groups.
- Loan Applications: AI-powered credit scoring systems might deny loans to individuals from underrepresented groups based on biased historical lending data.
Sources of Inaccuracy in Legal AI
Beyond bias, inaccuracies in legal AI can stem from several sources:
- Data Quality: AI models are only as good as the data they are trained on. Inaccurate, incomplete, or outdated legal data will inevitably lead to flawed outputs.
- Algorithm Limitations: Even with high-quality data, algorithms may struggle with the complexities and nuances of legal reasoning, leading to misinterpretations and incorrect predictions.
- Lack of Transparency: Many AI systems operate as "black boxes," making it difficult to understand how they arrive at their conclusions. This lack of transparency hinders the identification and correction of errors.
Mitigating Bias and Inaccuracy in Legal AI
Addressing bias and inaccuracy in Legal AI requires a multifaceted approach:
1. Data Auditing and Cleaning:
Thoroughly auditing and cleaning training datasets is paramount. This involves identifying and correcting biases, ensuring data completeness, and verifying data accuracy.
2. Algorithm Transparency and Explainability:
Developing more transparent and explainable AI models is crucial. This allows users to understand the reasoning behind the AI's outputs, identifying and correcting errors more effectively.
3. Diverse and Representative Datasets:
Training AI models on diverse and representative datasets is essential to mitigate bias. This ensures the AI system is exposed to a wider range of perspectives and experiences.
4. Human Oversight and Validation:
Human oversight remains crucial. Legal professionals should review and validate the AI's outputs, ensuring accuracy and fairness before making critical decisions.
5. Continuous Monitoring and Improvement:
Regular monitoring of AI systems for bias and inaccuracy is necessary. Continuous improvement through feedback loops and updates will help maintain accuracy and fairness over time.
The Future of Legal AI
The potential benefits of Legal AI are undeniable, but addressing bias and inaccuracy is crucial for responsible implementation. By proactively mitigating these risks, we can harness the power of AI to create a more efficient, equitable, and accurate legal system. The future of Legal AI depends on a commitment to ethical development and deployment.
Call to Action
Are you ready to navigate the ethical complexities of AI in the legal field? Engage in the discussion, share your thoughts, and let's work together to ensure responsible AI implementation in legal practices.
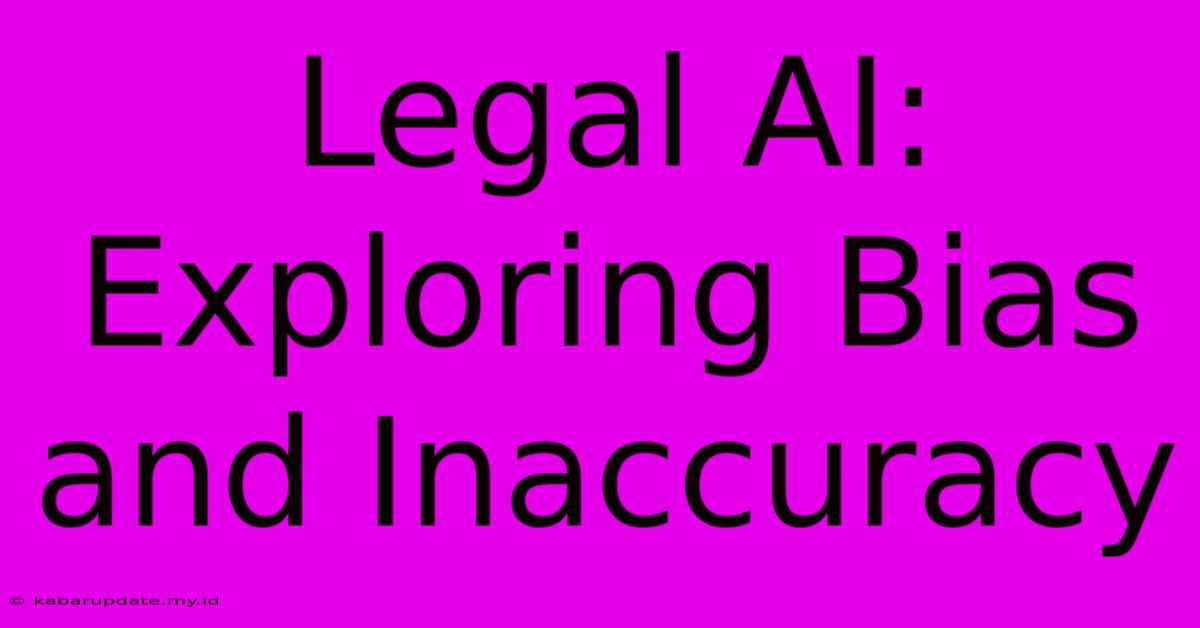
Thank you for taking the time to explore our website Legal AI: Exploring Bias And Inaccuracy. We hope you find the information useful. Feel free to contact us for any questions, and donβt forget to bookmark us for future visits!
We truly appreciate your visit to explore more about Legal AI: Exploring Bias And Inaccuracy. Let us know if you need further assistance. Be sure to bookmark this site and visit us again soon!
Featured Posts
-
Nowy Snape Fani Harry Ego Pottera Rozczarowani
Dec 13, 2024
-
Vyskazyvanie Bredli Zhyostkoe This Focuses On Bradleys Harsh Statement Making It Attractive For Users Searching For The Specific Nature Of The Controversy
Dec 13, 2024
-
Cba Przeszukuje Lux Veritatis
Dec 13, 2024
-
Es Jueves 5 De Diciembre 2024
Dec 13, 2024
-
Space Tourism 2024 2030 Outlook
Dec 13, 2024