La Dictadura Del Algoritmo: ¿Neutralidad O Sesgo?
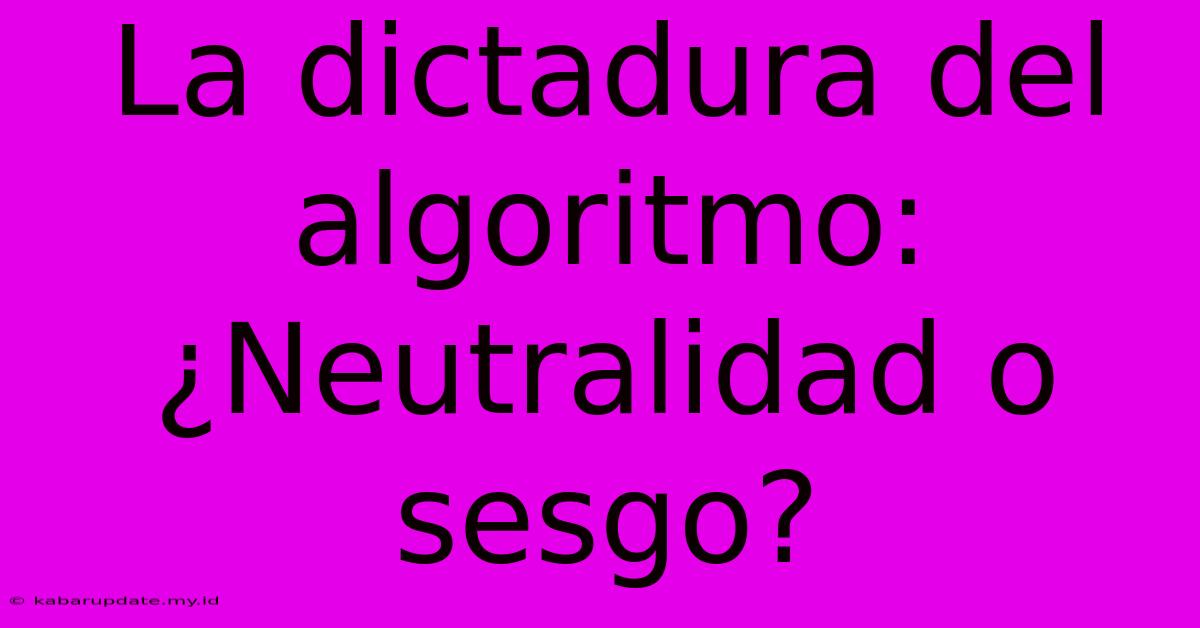
Discover more in-depth information on our site. Click the link below to dive deeper: Visit the Best Website meltwatermedia.ca. Make sure you don’t miss it!
Table of Contents
La Dictadura del Algoritmo: ¿Neutralidad o Sesgo?
The rise of algorithms has undeniably revolutionized our lives. From personalized recommendations on streaming services to curated news feeds, algorithms shape our daily experiences. But this pervasive influence raises a crucial question: are algorithms truly neutral, or do they harbor inherent biases that distort our realities? This article explores the complexities of algorithmic bias, its potential consequences, and what we can do to mitigate its effects.
The Illusion of Neutrality
Many believe algorithms, being based on mathematics and code, are objective and neutral. However, this perception is misleading. Algorithms are not created in a vacuum. They are designed by humans, reflecting their own biases, conscious or unconscious. These biases, embedded within the data used to train the algorithms and the choices made during their development, inevitably influence their outputs.
Data Bias: The Foundation of Algorithmic Inequality
Algorithms learn from data. If the data used to train an algorithm is biased – for example, reflecting existing societal prejudices against certain demographics – the algorithm will likely perpetuate and even amplify those biases. Consider a facial recognition system trained primarily on images of light-skinned individuals; it may perform poorly when identifying individuals with darker skin tones, leading to potentially discriminatory outcomes in law enforcement or security applications.
Algorithmic Design Choices: The Human Element
Even with unbiased data, the choices made during algorithm design can introduce bias. For instance, the selection of specific features to prioritize in a loan application algorithm can disproportionately affect certain applicant groups. Similarly, the parameters used to define “relevance” in a search engine can lead to the prioritization of certain viewpoints over others, shaping public discourse.
The Consequences of Algorithmic Bias
The consequences of algorithmic bias are far-reaching and can have significant societal impact:
- Discrimination: Biased algorithms can lead to unfair or discriminatory outcomes in areas like loan applications, hiring processes, and even criminal justice.
- Filter Bubbles and Echo Chambers: Personalized news feeds and recommendations can create "filter bubbles," isolating users from diverse perspectives and reinforcing pre-existing beliefs. This can lead to polarization and hinder informed decision-making.
- Erosion of Trust: When algorithms consistently produce biased results, it can erode public trust in technology and institutions.
Mitigating Algorithmic Bias: Towards a More Equitable Future
Addressing algorithmic bias requires a multi-faceted approach:
- Data Diversity and Representation: Ensuring the data used to train algorithms is diverse and representative of the population is crucial. This requires careful data collection and curation processes.
- Algorithmic Transparency and Explainability: Understanding how algorithms make decisions is essential for identifying and mitigating bias. Developments in explainable AI (XAI) are crucial in this regard.
- Independent Audits and Evaluation: Regular audits and evaluations of algorithms can help identify and address bias before it causes significant harm.
- Interdisciplinary Collaboration: Addressing algorithmic bias requires collaboration between computer scientists, social scientists, ethicists, and policymakers.
Practical Tips for Individuals
While large-scale changes require systemic effort, individuals can also play a role:
- Be Critical: Question the information presented to you online and consider diverse sources.
- Support Ethical AI Initiatives: Advocate for policies and practices that promote algorithmic fairness and transparency.
- Educate Yourself: Learn more about algorithmic bias and its implications.
Conclusion: The Path Forward
The "dictatorship of the algorithm" is a real concern. However, by acknowledging the existence of algorithmic bias and proactively addressing its causes, we can move towards a future where algorithms serve as tools for progress and equity rather than instruments of discrimination and division. The responsibility lies with developers, policymakers, and individuals alike to ensure algorithms are used responsibly and ethically. The future of fairness depends on it.
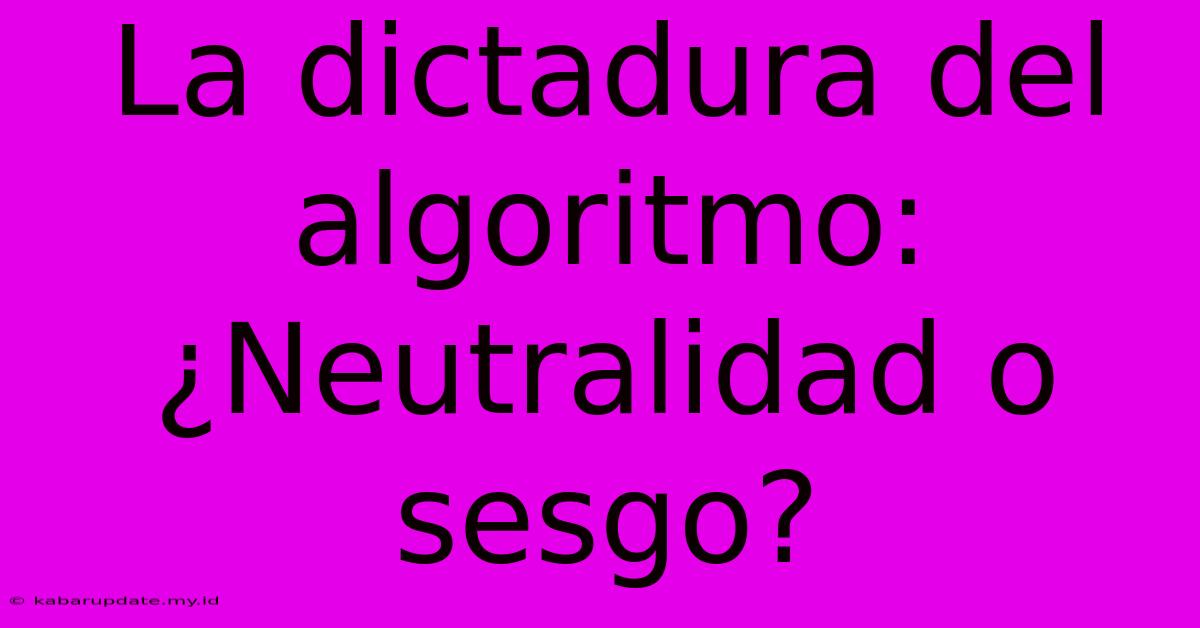
Thank you for taking the time to explore our website La Dictadura Del Algoritmo: ¿Neutralidad O Sesgo?. We hope you find the information useful. Feel free to contact us for any questions, and don’t forget to bookmark us for future visits!
We truly appreciate your visit to explore more about La Dictadura Del Algoritmo: ¿Neutralidad O Sesgo?. Let us know if you need further assistance. Be sure to bookmark this site and visit us again soon!
Featured Posts
-
Trump And Macron Tensions High In Talks
Dec 11, 2024
-
Redes Sociales La Ia Fomenta El Odio
Dec 11, 2024
-
Algoritmos Intenciones Humanas Resultados Sesgados
Dec 11, 2024
-
Filipinas 33 Afectados Por Carne De Tortuga
Dec 11, 2024
-
Predicciones Tigre 2025 Horoscopo Chino
Dec 11, 2024