¿Neutralidad Algorítmica? El Factor Humano
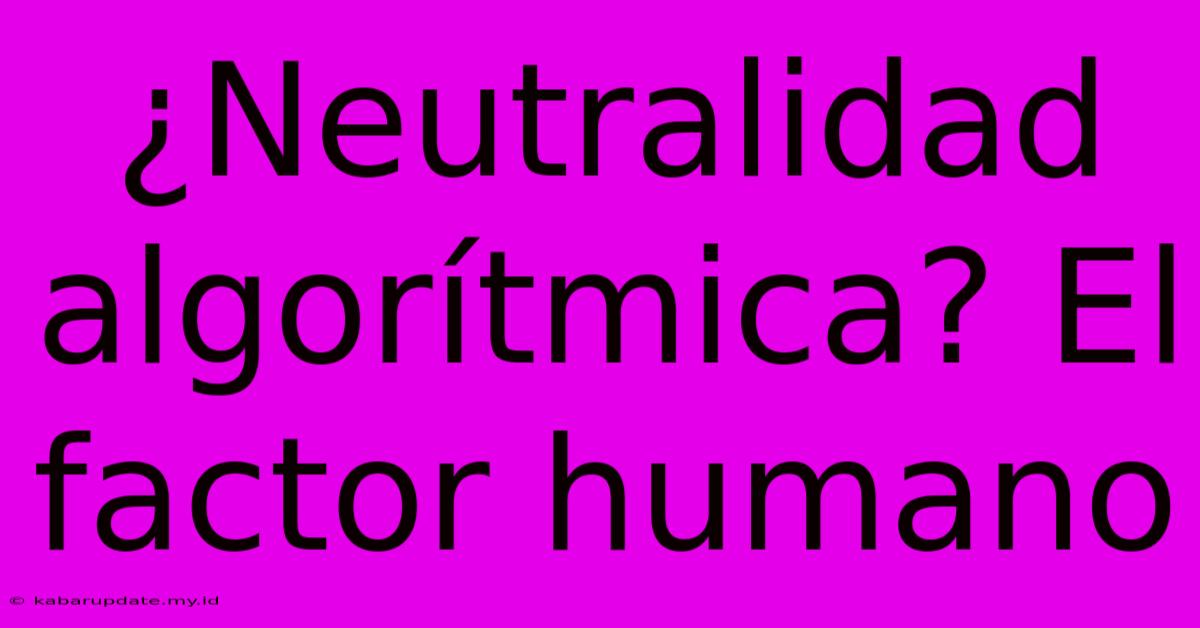
Discover more in-depth information on our site. Click the link below to dive deeper: Visit the Best Website meltwatermedia.ca. Make sure you don’t miss it!
Table of Contents
¿Neutralidad Algorítmica? El Factor Humano: Descifrando el Sesgo en la IA
The concept of algorithmic neutrality is often touted as a desirable goal in the development and deployment of artificial intelligence (IA). However, the reality is far more nuanced. While algorithms are designed to be objective, the human factor significantly influences their creation, implementation, and resulting outcomes, often introducing biases that undermine this ideal of neutrality. This article delves into the complexities of algorithmic neutrality, highlighting the crucial role of the human element in shaping seemingly impartial systems.
El Mito de la Neutralidad Pura
The idea of a completely neutral algorithm is, in many ways, a myth. Algorithms are built by humans, reflecting their perspectives, values, and inherent biases. These biases can be conscious or unconscious, subtle or overt, but they inevitably seep into the design and data used to train these systems. This leads to skewed results, perpetuating existing societal inequalities or even creating new ones.
For example, a facial recognition algorithm trained primarily on images of light-skinned individuals might perform poorly when identifying individuals with darker skin tones. This isn't due to an inherent flaw in the algorithm itself, but rather a bias introduced through the data used for training. The human choice to utilize a limited and non-representative dataset directly impacts the algorithm's performance and its consequent lack of neutrality.
Fuentes de Sesgo en la IA
Several factors contribute to the lack of algorithmic neutrality:
-
Datos sesgados: The data used to train AI systems often reflects existing societal biases. If the data contains disproportionate representations of certain groups, the algorithm will learn and perpetuate these biases. For example, datasets used in hiring algorithms may overrepresent certain demographic groups, leading to biased hiring practices.
-
Diseño sesgado: Even with unbiased data, the design choices made by developers can introduce bias. The selection of features, the weighting of variables, and the overall architecture of the algorithm can all influence the outcome, subtly favoring certain groups over others.
-
Interpretación sesgada: The interpretation of the results produced by an algorithm is also subject to human bias. Individuals may selectively focus on certain aspects of the output, ignoring or downplaying those that contradict their preconceived notions.
Mitigando el Sesgo Algorítmico: Pasos hacia la Equidad
Achieving true algorithmic neutrality is an ongoing challenge, but there are steps we can take to mitigate bias and promote fairness:
-
Diversidad en los equipos de desarrollo: Including diverse perspectives in the development process is crucial. Teams composed of individuals from various backgrounds and experiences are more likely to identify and address potential biases.
-
Datos representativos: Using representative datasets is fundamental. This requires careful consideration of data collection methods and a conscious effort to include data from all relevant groups.
-
Auditar algoritmos: Regularly auditing algorithms for bias is essential. This involves scrutinizing both the data and the algorithm's design to identify and correct potential sources of bias.
-
Transparencia y explicabilidad: Making algorithms more transparent and explainable allows for better understanding of their decision-making processes, facilitating the identification and mitigation of bias.
Conclusión: Un Futuro más Justo a través de la Conciencia
Algorithmic neutrality is not a given; it's an aspiration that requires constant vigilance and proactive effort. By acknowledging the significant role of the human factor in shaping AI systems, we can work towards creating more equitable and just technologies. This requires a collective commitment to diversity, transparency, and rigorous auditing of algorithms. The future of AI depends on our ability to consciously address and mitigate the biases that threaten to undermine its potential for good. Let's strive for a future where algorithms truly serve all of humanity, not just a select few.
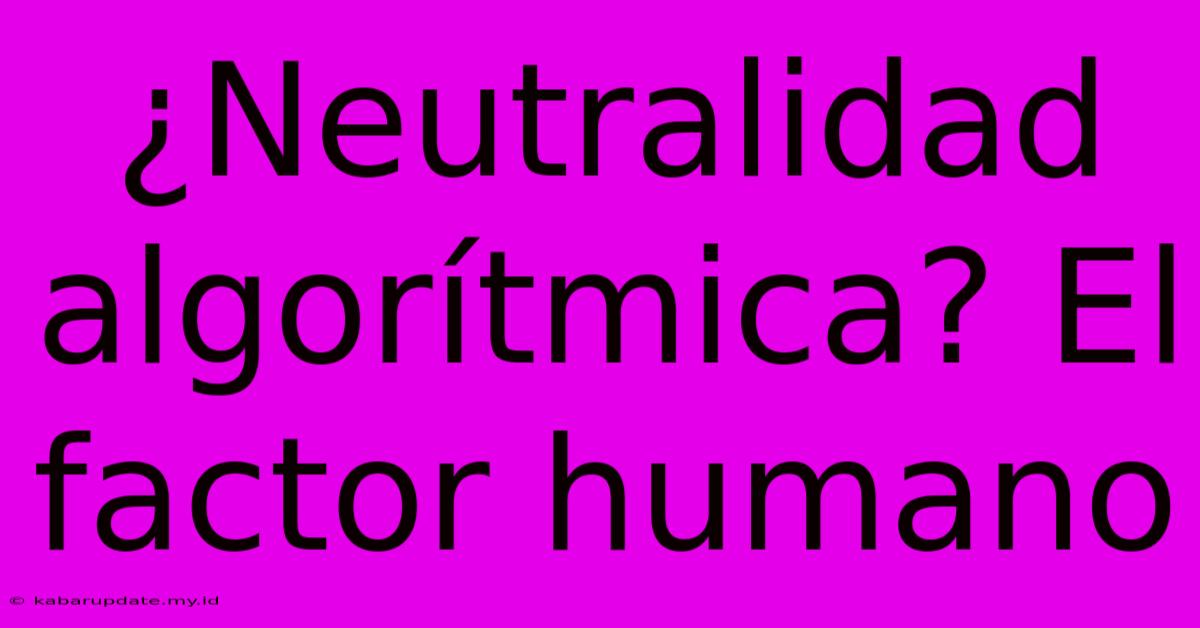
Thank you for taking the time to explore our website ¿Neutralidad Algorítmica? El Factor Humano. We hope you find the information useful. Feel free to contact us for any questions, and don’t forget to bookmark us for future visits!
We truly appreciate your visit to explore more about ¿Neutralidad Algorítmica? El Factor Humano. Let us know if you need further assistance. Be sure to bookmark this site and visit us again soon!
Featured Posts
-
Trece Al Dia Infancia Segura 25 Nov 2024
Dec 11, 2024
-
Dia Del Voluntariado Entrega Y Servicio
Dec 11, 2024
-
Cyclone Abort Plane Turns Around
Dec 11, 2024
-
Chino 2025 Que Signos Tendran Exito
Dec 11, 2024
-
United Healthcare Ceo Murdered
Dec 11, 2024