Mitigating AI Bias In Legal Gender Balance
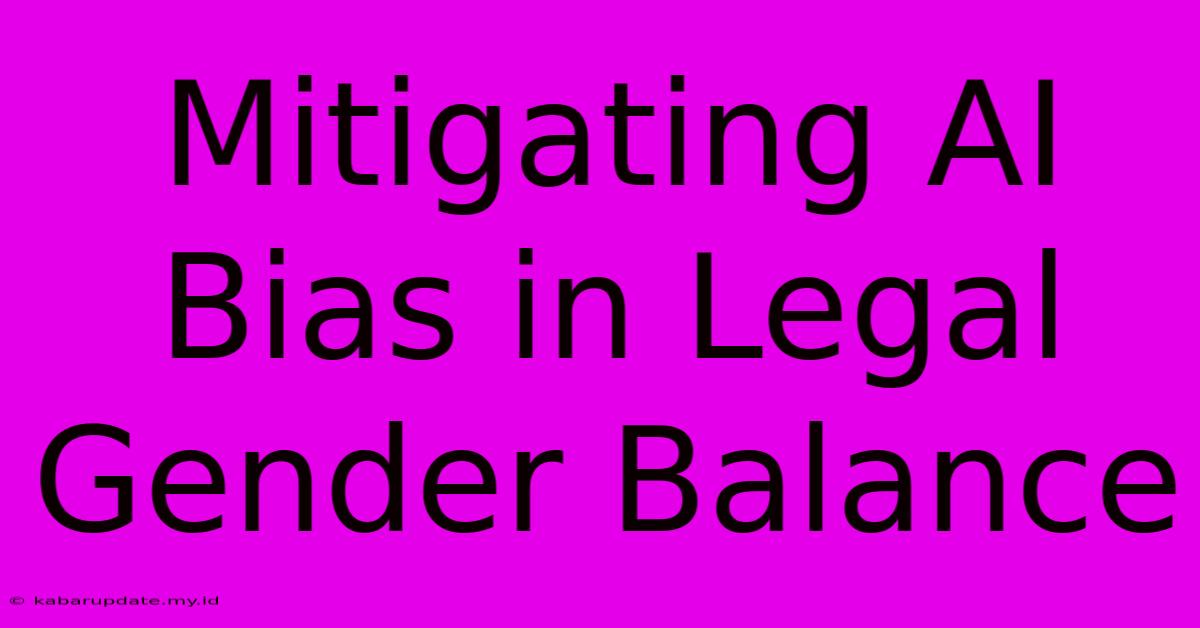
Discover more in-depth information on our site. Click the link below to dive deeper: Visit the Best Website meltwatermedia.ca. Make sure you donβt miss it!
Table of Contents
Mitigating AI Bias in Legal Gender Balance
Achieving true gender balance in the legal profession is a complex challenge, and Artificial Intelligence (AI) β while offering potential solutions β can inadvertently exacerbate existing biases. This article explores the crucial issue of AI bias in legal gender balance and offers practical strategies for mitigation.
Understanding the Problem: AI Bias and its Impact
AI systems, trained on historical data often reflecting societal biases, can perpetuate and even amplify gender inequality within the legal field. This bias can manifest in several ways:
1. Recruitment and Hiring:
AI-powered recruitment tools, trained on data showing historical gender imbalances in hiring, might unfairly rank female candidates lower than similarly qualified male candidates. This reinforces existing disparities and prevents a diverse and representative workforce.
2. Case Outcomes:
Algorithmic bias in legal tech can skew case outcomes. For example, an AI system trained on data where judges have historically favored male plaintiffs could lead to biased predictions and decisions, further disadvantaging women.
3. Career Progression:
AI systems used to assess performance and identify high-potential employees may inadvertently overlook the contributions of women, perpetuating the "glass ceiling" effect and hindering career advancement.
Strategies for Mitigating AI Bias
Addressing AI bias requires a multi-pronged approach involving careful data selection, algorithm design, and ongoing monitoring.
1. Data Diversity and Preprocessing:
The foundation of fair AI lies in diverse and representative data. Before training an AI system, it's crucial to:
- Identify and address historical biases: Carefully examine the dataset for gender imbalances and consciously correct them. This might involve oversampling underrepresented groups or using techniques like re-weighting.
- Ensure data quality: Inaccurate or incomplete data will only amplify existing biases. Thorough data cleaning and validation are essential.
- Consider intersectionality: Recognize that gender bias interacts with other factors like race and socioeconomic status. The dataset should reflect this complexity.
2. Algorithmic Transparency and Explainability:
Understanding how an AI system arrives at its conclusions is vital for identifying and correcting bias. Prioritize:
- Explainable AI (XAI): Choose algorithms that offer insights into their decision-making process, allowing for easier identification of potential biases.
- Regular audits: Conduct periodic audits of the AI system to assess its fairness and identify areas for improvement. Involve diverse teams in these audits.
3. Human Oversight and Intervention:
AI should be seen as a tool to augment human judgment, not replace it. Maintain:
- Human-in-the-loop systems: Incorporate human review in critical decision-making processes to override potentially biased AI recommendations.
- Bias awareness training: Educate legal professionals on AI bias and its potential impact to foster critical evaluation of AI-generated insights.
4. Continuous Monitoring and Improvement:
Bias mitigation is an ongoing process, not a one-time fix. Establish:
- Performance metrics: Regularly track key metrics related to gender equity to monitor the effectiveness of bias mitigation strategies.
- Feedback mechanisms: Create systems for collecting feedback from users on the AI system's performance, identifying and addressing any concerns about bias.
Conclusion: Towards a Fairer Future
Mitigating AI bias in legal gender balance is not merely a technical challenge; it's a crucial step towards achieving a fairer and more equitable legal profession. By implementing the strategies outlined above, legal organizations can leverage the power of AI while safeguarding against the perpetuation of harmful gender biases. The future of AI in law depends on our commitment to responsible development and deployment. Let's work collaboratively to build AI systems that promote, rather than hinder, gender equality.
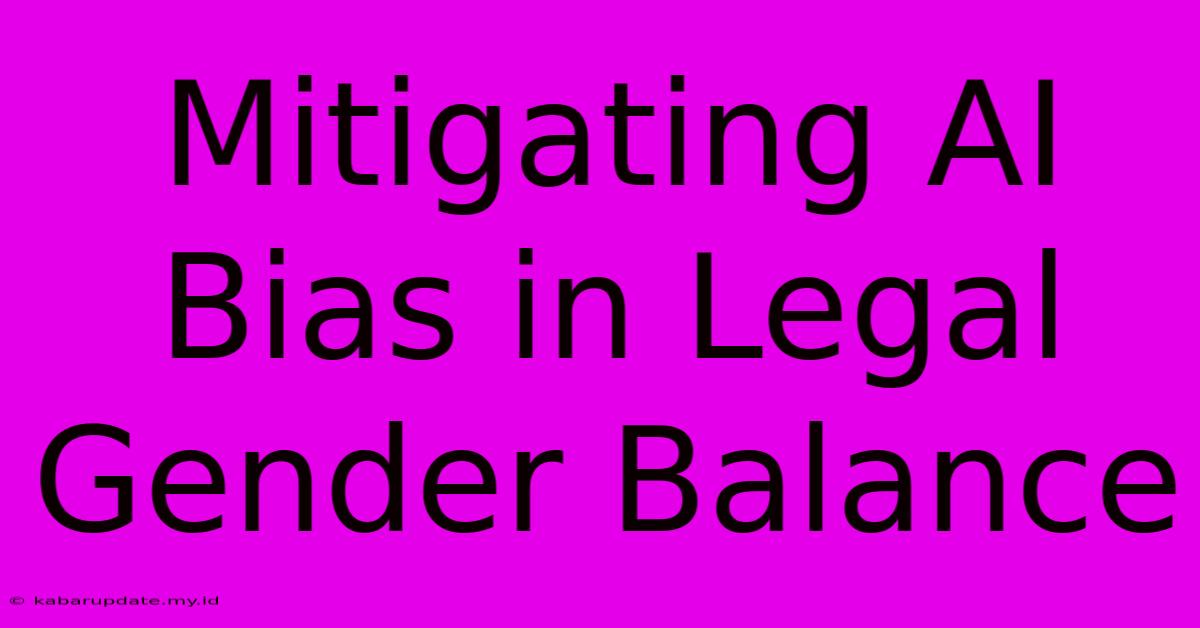
Thank you for taking the time to explore our website Mitigating AI Bias In Legal Gender Balance. We hope you find the information useful. Feel free to contact us for any questions, and donβt forget to bookmark us for future visits!
We truly appreciate your visit to explore more about Mitigating AI Bias In Legal Gender Balance. Let us know if you need further assistance. Be sure to bookmark this site and visit us again soon!
Featured Posts
-
Alverca Vence Vizela Por 4 2
Dec 13, 2024
-
Star Wars Skeleton Crew Details
Dec 13, 2024
-
Angliyskaya Premer Liga Fulkhem Brayton Polniy Analiz Matcha
Dec 13, 2024
-
Two Arrested In Chennai Airport Turtle Trafficking
Dec 13, 2024
-
Space Tourism Leisure Travel Expands
Dec 13, 2024