Legal Tech: Addressing AI Gender Bias
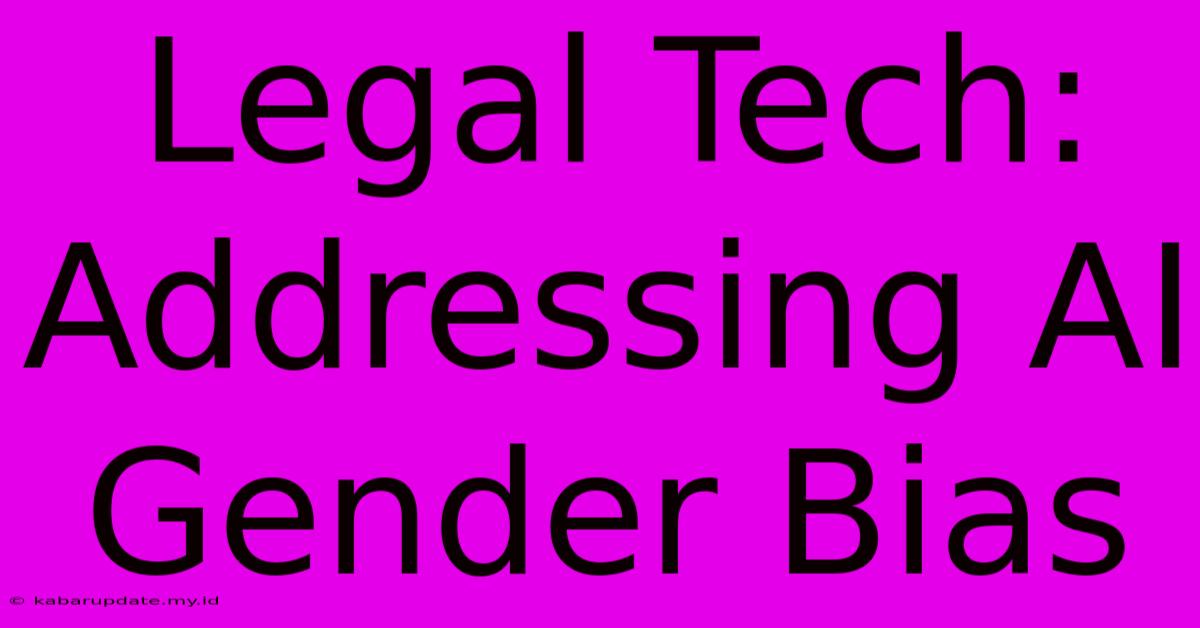
Discover more in-depth information on our site. Click the link below to dive deeper: Visit the Best Website meltwatermedia.ca. Make sure you donβt miss it!
Table of Contents
Legal Tech: Addressing AI Gender Bias
The rise of Artificial Intelligence (AI) in legal tech presents incredible opportunities for increased efficiency and accuracy. However, a significant challenge looms: the potential for AI systems to perpetuate and even amplify existing gender biases. This article explores the issue of AI gender bias in the legal field and offers practical strategies for mitigation.
The Problem: AI Bias in Legal Tech
AI algorithms are trained on data, and if that data reflects societal biases β such as underrepresentation of women in leadership roles or skewed sentencing patterns β the AI system will likely replicate and even exacerbate these biases. This has serious consequences in legal applications.
Examples of AI Bias in Legal Settings:
- Predictive Policing: Algorithms used to predict crime hotspots might disproportionately target areas with higher female poverty rates, reinforcing existing societal inequalities.
- Sentencing Recommendations: AI systems advising on sentencing could inadvertently recommend harsher penalties for female defendants compared to male defendants with similar backgrounds, due to biased training data.
- Contract Analysis: AI reviewing contracts might miss crucial clauses impacting women's rights or fail to recognize gendered language subtly disadvantaging female parties.
- Recruitment and Promotion: AI-powered recruitment tools could filter out qualified female candidates based on biased data reflecting historical hiring practices.
Addressing the Bias: Mitigation Strategies
The good news is that we can actively address AI gender bias. It requires a multi-pronged approach focusing on data, algorithms, and human oversight.
1. Data Diversity and Quality:
- Diverse Datasets: Training AI on datasets that accurately reflect the diversity of the population is crucial. This means actively seeking and incorporating data representing women from various backgrounds and experiences.
- Data Auditing: Regularly audit datasets for biases. Look for discrepancies in representation and systematically correct for imbalances. Tools exist to help detect skewed datasets.
- Bias Detection Techniques: Employ techniques that identify and quantify biases within datasets, enabling proactive correction before training the AI model.
2. Algorithm Design and Transparency:
- Explainable AI (XAI): Use XAI techniques to understand how AI algorithms arrive at their conclusions. This transparency helps identify potential biases embedded in the decision-making process.
- Fairness-Aware Algorithms: Develop and utilize algorithms specifically designed to minimize bias. These algorithms incorporate fairness constraints during training.
- Algorithmic Audits: Conduct regular audits of algorithms to assess their fairness and identify potential points of bias.
3. Human Oversight and Accountability:
- Human-in-the-Loop Systems: Integrate human oversight into the AI system. This allows human experts to review AI recommendations and intervene when necessary, correcting for bias.
- Ethical Guidelines and Regulations: Develop and enforce ethical guidelines and regulations for the development and deployment of AI in legal settings. This requires collaborative efforts from legal professionals, tech developers, and policymakers.
- Ongoing Training and Education: Educate legal professionals and tech developers on the issue of AI bias and best practices for mitigation.
The Future of Fair AI in Legal Tech
Eliminating AI bias is an ongoing process requiring constant vigilance and adaptation. By prioritizing data diversity, transparent algorithms, and robust human oversight, the legal tech industry can harness the power of AI while mitigating the risks of perpetuating harmful gender biases. This ensures a fairer and more equitable legal system for all.
Call to Action
Are you a legal professional or tech developer working with AI? Share your experiences and strategies for mitigating bias in the comments below. Let's collaborate to build a more inclusive and equitable future for legal tech.
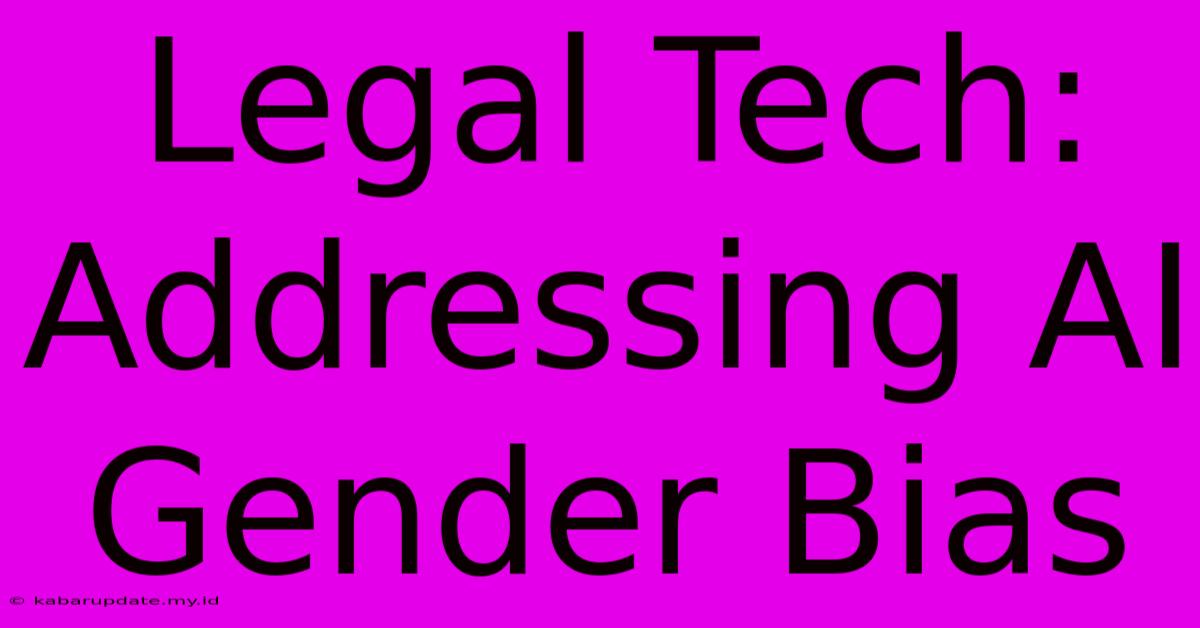
Thank you for taking the time to explore our website Legal Tech: Addressing AI Gender Bias. We hope you find the information useful. Feel free to contact us for any questions, and donβt forget to bookmark us for future visits!
We truly appreciate your visit to explore more about Legal Tech: Addressing AI Gender Bias. Let us know if you need further assistance. Be sure to bookmark this site and visit us again soon!
Featured Posts
-
Triathlon Ends In 3 Way Tie
Dec 13, 2024
-
Buenos Aires Trap Dos Jornadas Y Mas
Dec 13, 2024
-
Serial Harry Potter Pierwszy Zwiastun
Dec 13, 2024
-
Closing The Ai Implementation Gap
Dec 13, 2024
-
Airport Turtle Seizure Chennai
Dec 13, 2024