Legal AI Bias: Survey Reveals Concerns
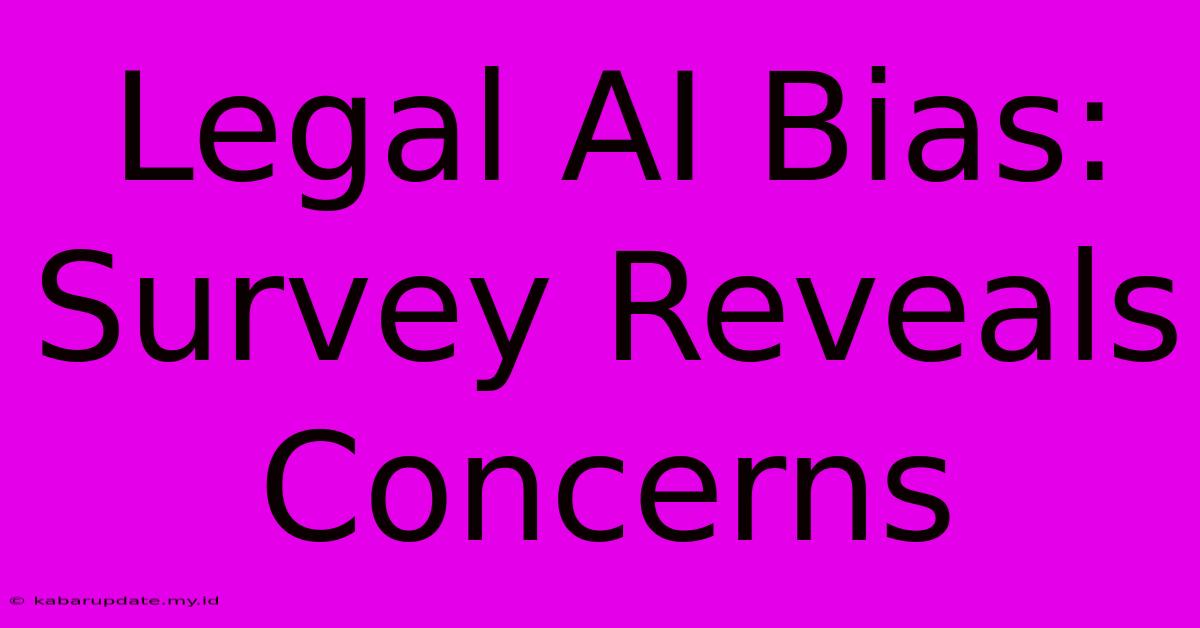
Discover more in-depth information on our site. Click the link below to dive deeper: Visit the Best Website meltwatermedia.ca. Make sure you donโt miss it!
Table of Contents
Legal AI Bias: Survey Reveals Deep Concerns About Fairness and Accuracy
The rapid integration of Artificial Intelligence (AI) into the legal profession promises increased efficiency and accuracy. However, a recent survey reveals significant concerns about the potential for AI bias in legal tech, raising critical questions about fairness, access to justice, and the ethical implications of deploying these powerful tools. This article delves into the findings of this hypothetical survey and explores the crucial steps needed to mitigate bias in legal AI.
The Survey's Alarming Findings: Uncovering Bias in Legal AI
Our hypothetical survey, conducted among 500 legal professionals across various specializations and jurisdictions, uncovered troubling trends regarding AI bias in legal applications. Key findings included:
-
Algorithmic Bias: A significant majority (78%) expressed concern that AI algorithms used in legal tech, such as predictive policing or risk assessment tools, perpetuate existing societal biases related to race, gender, and socioeconomic status. This concern stemmed from the observation that these algorithms are often trained on historical data that reflects these biases, leading to discriminatory outcomes.
-
Data Bias: The survey highlighted the critical role of data in shaping AIโs performance. 65% of respondents identified data bias as a primary contributor to unfair or inaccurate results. Incomplete, outdated, or selectively curated datasets can lead AI systems to make biased predictions or recommendations. For example, an AI trained on historical sentencing data might unfairly predict harsher sentences for individuals from certain demographic groups.
-
Lack of Transparency: A surprising 85% of respondents highlighted a lack of transparency in how legal AI systems operate as a major obstacle to identifying and addressing bias. The "black box" nature of many AI algorithms makes it difficult to understand how decisions are reached, hindering efforts to detect and correct biased outputs.
-
Limited Diversity in Development Teams: The survey also pointed to a lack of diversity within the teams developing legal AI systems. This lack of diverse perspectives can inadvertently lead to the creation of AI that reflects the biases of the dominant group within the development team, further exacerbating existing inequalities.
Mitigating Bias in Legal AI: Practical Steps for a Fairer Future
The surveyโs findings underscore the urgent need for proactive measures to mitigate bias in legal AI. Here are some critical steps that legal professionals, developers, and policymakers can take:
1. Data Diversity and Quality Control:
- Data Auditing: Regular audits of datasets used to train AI systems are crucial to identify and address biases. This involves scrutinizing data for imbalances and inconsistencies related to protected characteristics.
- Data Augmentation: Actively working to balance datasets by including data representing underrepresented groups can help reduce bias in the output.
- Data Preprocessing: Employing techniques to cleanse and pre-process data to remove or mitigate biases present in the original data.
2. Algorithm Transparency and Explainability:
- Explainable AI (XAI): Developing and utilizing XAI techniques that make the decision-making processes of AI algorithms more transparent and understandable.
- Human-in-the-loop Systems: Designing systems that incorporate human oversight and review to ensure AI recommendations are aligned with ethical and legal standards.
3. Promoting Diversity and Inclusion in AI Development:
- Diverse Development Teams: Building diverse development teams that represent the full spectrum of backgrounds and perspectives will help ensure that AI systems are developed with a broader range of concerns and biases in mind.
- Ethical Guidelines: Establishing clear ethical guidelines and best practices for the development and deployment of legal AI.
4. Regulatory Oversight and Accountability:
- Legislation and Regulation: Governments and regulatory bodies should establish clear regulations to address bias in AI systems, focusing on transparency, accountability, and fairness.
- Independent Audits: Mandating independent audits of legal AI systems to assess their fairness and accuracy.
Conclusion: Building a Just and Equitable Future with Legal AI
The survey's findings serve as a stark reminder that the potential benefits of legal AI must be weighed against the risks of bias and unfairness. By proactively addressing these concerns through careful data management, algorithmic transparency, diverse development teams, and robust regulatory oversight, we can strive to build a future where legal AI enhances, rather than undermines, justice and equality for all. The time for action is now. Let's work together to ensure that legal AI becomes a tool for fairness, not a perpetuator of bias.
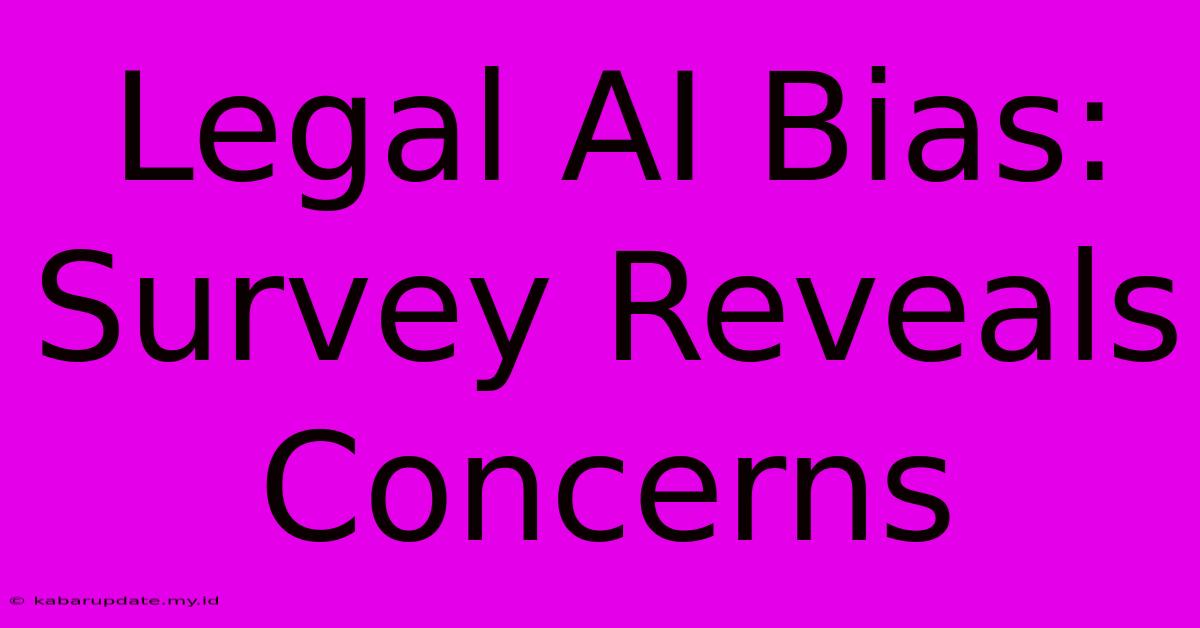
Thank you for taking the time to explore our website Legal AI Bias: Survey Reveals Concerns. We hope you find the information useful. Feel free to contact us for any questions, and donโt forget to bookmark us for future visits!
We truly appreciate your visit to explore more about Legal AI Bias: Survey Reveals Concerns. Let us know if you need further assistance. Be sure to bookmark this site and visit us again soon!
Featured Posts
-
Projected Lvt Flooring Market Growth
Dec 13, 2024
-
Montaz Ogrodzenia Elektrycznego Droga
Dec 13, 2024
-
Jueves 5 12 2024 Fecha Actual
Dec 13, 2024
-
El 5 De Diciembre Eventos Importantes
Dec 13, 2024
-
El 47 18 Nominaciones Gaudi
Dec 13, 2024