Legal AI: A Survey On Bias And Errors
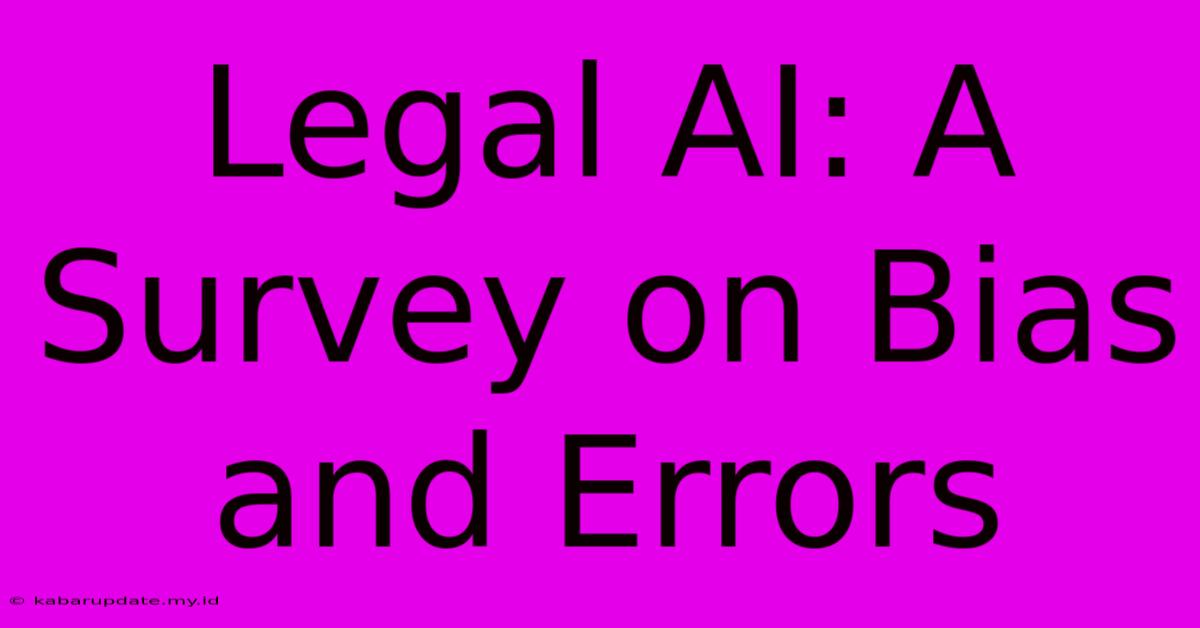
Discover more in-depth information on our site. Click the link below to dive deeper: Visit the Best Website meltwatermedia.ca. Make sure you donβt miss it!
Table of Contents
Legal AI: A Survey on Bias and Errors
The burgeoning field of Legal AI promises to revolutionize the legal profession, automating tasks, enhancing efficiency, and potentially improving access to justice. However, the deployment of AI in legal settings is not without significant challenges. This article surveys the prevalent issues of bias and errors in Legal AI systems and explores strategies for mitigation.
The Sources of Bias in Legal AI
Bias in Legal AI stems from various sources, all ultimately tracing back to the data used to train these systems. This data, often sourced from historical legal records and practices, may reflect existing societal biases related to race, gender, socioeconomic status, and other protected characteristics.
1. Data Bias: The Foundation of the Problem
The most significant source of bias lies in the training data itself. If the data used to train an AI model disproportionately represents certain demographics or viewpoints, the model will inevitably learn and perpetuate those biases. For instance, an AI system trained on case data reflecting historical gender disparities in sentencing may predict harsher sentences for female defendants, even if the current legal framework mandates gender-neutral sentencing.
2. Algorithmic Bias: The Amplification Effect
Even with unbiased data, algorithmic bias can creep in. The algorithms themselves, the mathematical models used to process the data, can inadvertently amplify or create new biases. This can occur due to design flaws or limitations in the algorithm's ability to handle complex and nuanced legal situations.
3. Feedback Loops and Bias Reinforcement
AI systems are not static; they often learn and adapt through feedback loops. If the outputs of a biased AI system are used to inform further decisions, this can create a self-reinforcing cycle, perpetuating and amplifying existing biases over time. This is particularly concerning in legal contexts where decisions have significant real-world consequences.
Types of Errors in Legal AI
Beyond bias, Legal AI systems are susceptible to various errors, many stemming from their limitations in understanding context and nuance.
1. Factual Errors: Misinterpreting Information
Legal AI systems rely on accurate and complete data. Errors in the data, inconsistencies in formatting, or ambiguities in language can lead to factual inaccuracies in the AI's output. This can range from misinterpreting specific clauses in a contract to incorrectly identifying relevant precedents.
2. Logical Errors: Faulty Reasoning
Even with accurate data, an AI system may reach incorrect conclusions due to limitations in its reasoning capabilities. It may fail to consider relevant exceptions, overlook crucial details, or make incorrect inferences based on incomplete information. This is particularly problematic in areas requiring sophisticated legal reasoning, such as contract interpretation or case analysis.
3. Interpretability Issues: The "Black Box" Problem
Many AI systems, particularly deep learning models, operate as "black boxes," making it difficult to understand how they arrive at their conclusions. This lack of transparency makes it challenging to identify and correct errors or biases, hindering accountability and trust.
Mitigating Bias and Errors in Legal AI
Addressing bias and errors in Legal AI requires a multi-faceted approach:
- Data Auditing and Preprocessing: Carefully examining training data for biases and pre-processing it to mitigate these biases is crucial. This includes techniques like data augmentation to increase representation of underrepresented groups.
- Algorithm Selection and Evaluation: Choosing algorithms less prone to bias and rigorously evaluating their performance on diverse datasets are essential steps.
- Explainable AI (XAI): Implementing XAI techniques to make the decision-making process of AI systems more transparent and understandable can improve accountability and trust.
- Human Oversight and Review: Incorporating human review in the AI's decision-making process is crucial to catch errors and biases that the AI might miss.
- Continuous Monitoring and Improvement: Regularly monitoring the performance of Legal AI systems and actively seeking feedback to identify and address issues are key to ensuring ongoing accuracy and fairness.
Conclusion
Legal AI holds immense potential to transform the legal field, but its ethical deployment requires careful consideration of bias and error mitigation strategies. By proactively addressing these challenges, we can harness the power of AI to create a more efficient, equitable, and just legal system. The ongoing development and refinement of Legal AI must prioritize fairness, transparency, and accountability to ensure its beneficial integration into legal practice.
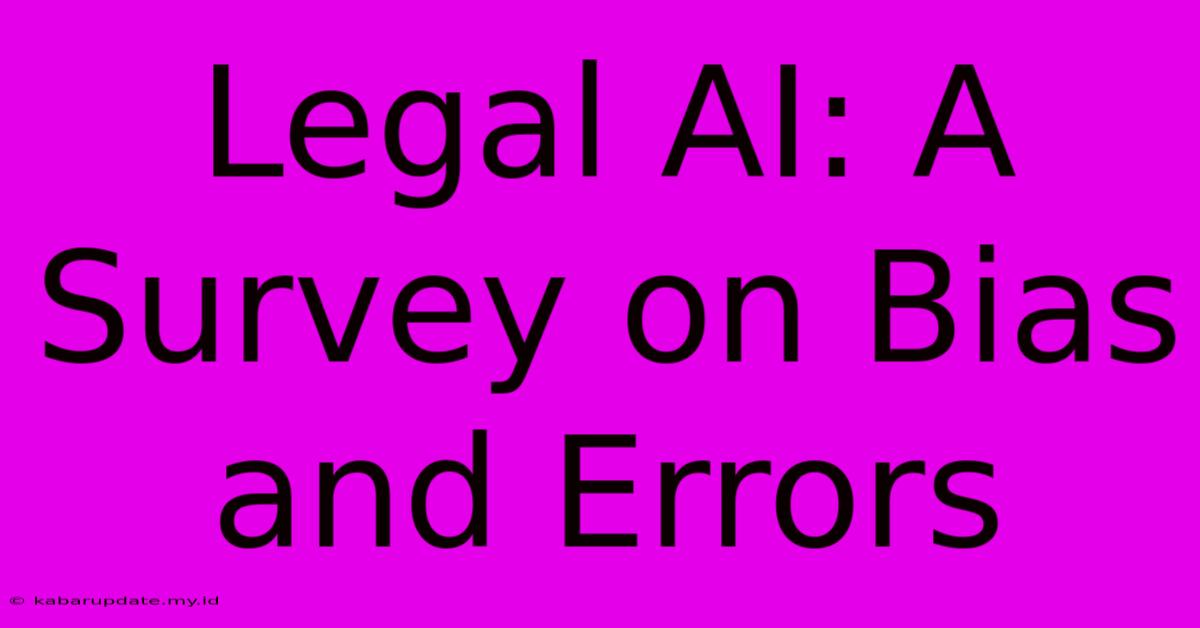
Thank you for taking the time to explore our website Legal AI: A Survey On Bias And Errors. We hope you find the information useful. Feel free to contact us for any questions, and donβt forget to bookmark us for future visits!
We truly appreciate your visit to explore more about Legal AI: A Survey On Bias And Errors. Let us know if you need further assistance. Be sure to bookmark this site and visit us again soon!
Featured Posts
-
Van Der Vaarts Onthulling Alcohol Op Training
Dec 13, 2024
-
Three Way Dead Heat Race Report
Dec 13, 2024
-
Ireland Weather Warnings Issued
Dec 13, 2024
-
Chennai Foils Turtle Smuggling Ring
Dec 13, 2024
-
Rozwod Harry Ego Nowe Zycie Poza Usa
Dec 13, 2024