Fengal Cyclone: Tracking And Prediction Issues
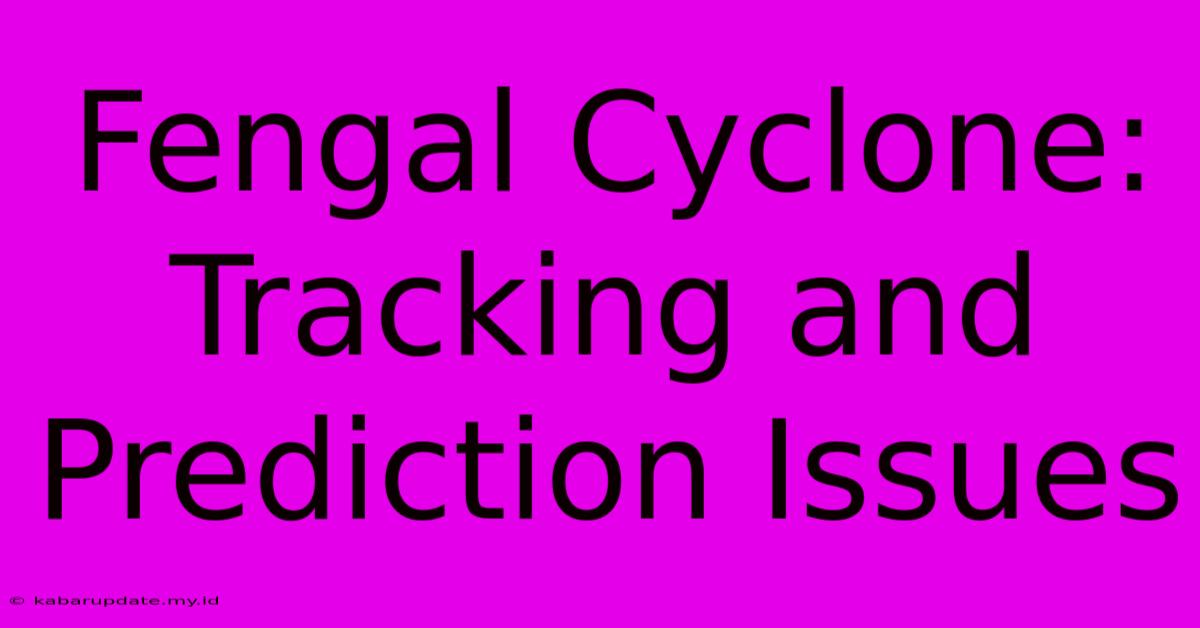
Discover more in-depth information on our site. Click the link below to dive deeper: Visit the Best Website meltwatermedia.ca. Make sure you donβt miss it!
Table of Contents
Fengal Cyclone: Tracking and Prediction Issues
The unpredictable nature of cyclones presents significant challenges to meteorological agencies worldwide. The Fengal Cyclone, a hypothetical example used for illustrative purposes, highlights these difficulties in tracking and prediction. While Fengal isn't a real cyclone, the challenges it represents are very real and experienced with actual tropical cyclones every year. This article explores the key issues involved in accurately tracking and predicting cyclone trajectories and intensity.
Challenges in Cyclone Tracking
Accurately tracking a cyclone's path relies heavily on a combination of observational data and sophisticated numerical weather prediction (NWP) models. However, several factors can significantly impact the accuracy of tracking:
Data Scarcity in Remote Regions
Many cyclones form and intensify over vast ocean expanses lacking sufficient observational infrastructure. Sparse data from weather buoys, ships, and sparsely populated islands hinder the initial detection and accurate assessment of a cyclone's strength and movement. This data scarcity is particularly problematic for cyclones like a hypothetical Fengal, forming far from land-based weather stations.
Rapid Intensification
Cyclones can undergo periods of rapid intensification (RI), where their wind speeds increase dramatically in a short time. Predicting RI is notoriously difficult because subtle changes in atmospheric conditions can trigger rapid intensification, often exceeding the capabilities of current prediction models. A rapidly intensifying Fengal cyclone could easily outpace prediction models, leading to inaccurate forecasts and insufficient warning times.
Interaction with Other Weather Systems
Cyclones don't exist in isolation. Their interaction with other weather systems, such as upper-level troughs or high-pressure systems, can significantly alter their trajectory and intensity. Accurately predicting these complex interactions presents a significant challenge. A hypothetical Fengal cyclone's path might be unexpectedly diverted or weakened by an unforeseen interaction with a neighboring weather system.
Limitations of Prediction Models
Numerical weather prediction models, though highly sophisticated, are not perfect. Several limitations impact the accuracy of cyclone predictions:
Model Resolution and Physics
The accuracy of NWP models depends on their resolution (the grid size used to represent the atmosphere) and the physical processes they represent. Higher resolution models offer greater detail but require significantly more computational power. Limitations in model physics, particularly in representing the complex processes within a cyclone, can lead to inaccuracies in intensity and track forecasts.
Initial Condition Uncertainty
Slight inaccuracies in the initial atmospheric conditions used to run the models can lead to significant differences in the predicted cyclone track and intensity over time. This is known as the "butterfly effect," where small changes in initial conditions can have large-scale consequences. The uncertainty in initial conditions significantly limits the skill of long-range forecasts for a cyclone like a hypothetical Fengal.
Ensemble Forecasting
To account for initial condition uncertainty, meteorologists use ensemble forecasting, running multiple model simulations with slightly different initial conditions. By analyzing the spread of the ensemble forecasts, meteorologists can assess the uncertainty associated with the predictions. However, even ensemble forecasts may not capture all possible outcomes for a complex system like a cyclone.
Improving Cyclone Tracking and Prediction
Improving cyclone tracking and prediction requires a multifaceted approach:
- Enhanced Observational Networks: Investing in more advanced observing systems, including satellites, weather buoys, and unmanned aerial vehicles (UAVs), is crucial for improving data coverage, especially over remote ocean areas.
- Improved Model Physics: Continued research into improving the physical representations within NWP models, particularly those relating to the processes that govern cyclone intensification, is vital.
- Advanced Data Assimilation Techniques: More sophisticated data assimilation techniques can better integrate diverse observational data into NWP models, leading to more accurate initial conditions and improved forecasts.
- Community Collaboration: International collaboration and data sharing among meteorological agencies are crucial for improving global cyclone forecasting capabilities.
By addressing these challenges, the meteorological community strives to improve the accuracy and timeliness of cyclone predictions, ultimately saving lives and mitigating the devastating impacts of these powerful storms. Improved prediction will be vital for effective disaster preparedness and response in the face of events like our hypothetical Fengal Cyclone.
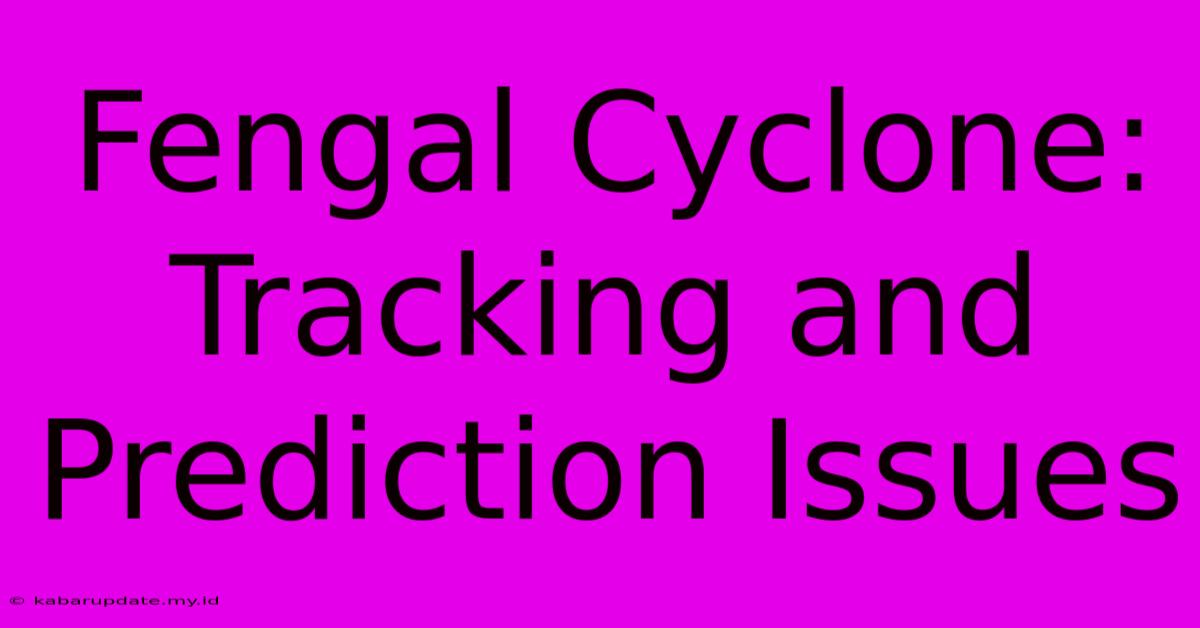
Thank you for taking the time to explore our website Fengal Cyclone: Tracking And Prediction Issues. We hope you find the information useful. Feel free to contact us for any questions, and donβt forget to bookmark us for future visits!
We truly appreciate your visit to explore more about Fengal Cyclone: Tracking And Prediction Issues. Let us know if you need further assistance. Be sure to bookmark this site and visit us again soon!
Featured Posts
-
United Healthcare Ceo Death New York Shooting
Dec 11, 2024
-
France Us Ties Tested Trump Macron Talks
Dec 11, 2024
-
25 De Noviembre Dia De La Infancia
Dec 11, 2024
-
Fengal Cyclone Claims 19 Lives In South Asia
Dec 11, 2024
-
Rmg Superando Retos En Misiones Salesianas
Dec 11, 2024