Bias And Inaccuracy In Legal AI: Survey Data
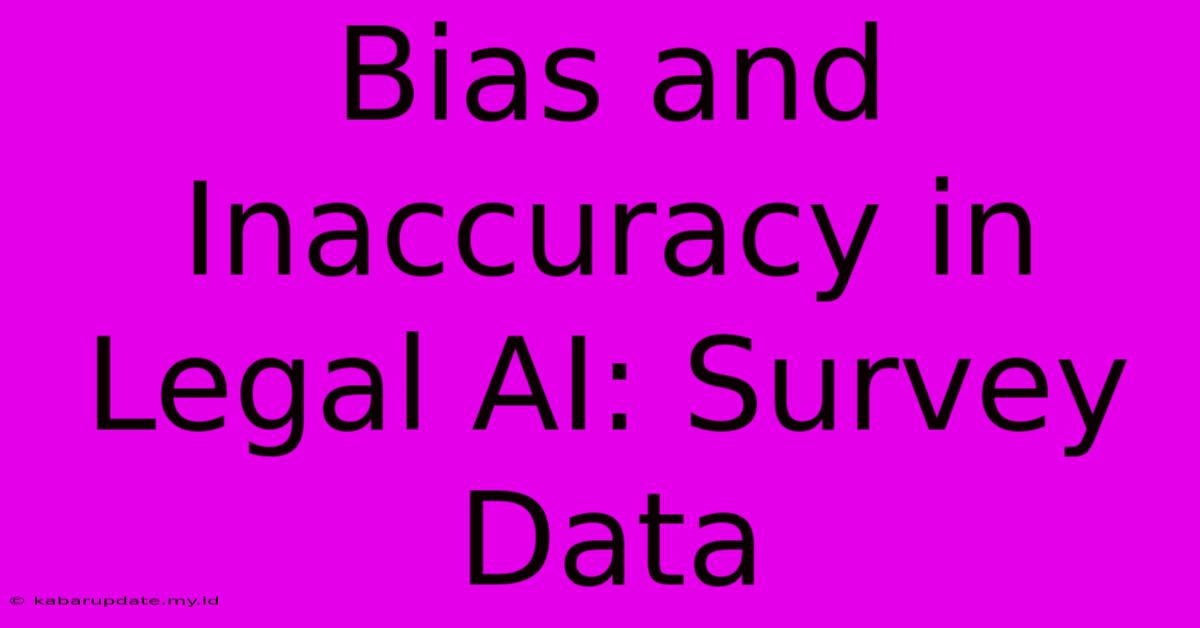
Discover more in-depth information on our site. Click the link below to dive deeper: Visit the Best Website meltwatermedia.ca. Make sure you donβt miss it!
Table of Contents
Bias and Inaccuracy in Legal AI: Survey Data Reveals Concerning Trends
The rapid advancement of Artificial Intelligence (AI) is transforming numerous sectors, and the legal field is no exception. However, the integration of AI in legal processes isn't without its challenges. A growing body of research highlights significant concerns regarding bias and inaccuracy in legal AI systems. This article delves into survey data revealing these concerning trends, exploring their implications and offering potential solutions.
The Prevalence of Bias in Legal AI
Numerous surveys and studies consistently point to the presence of bias within legal AI tools. This bias often stems from the data used to train these algorithms. If the training data reflects existing societal biases β such as racial, gender, or socioeconomic disparities β the AI system will likely perpetuate and even amplify these biases in its outputs.
Examples of Bias in Action:
- Predictive policing algorithms: Studies have shown that algorithms used to predict crime recidivism often exhibit racial bias, disproportionately targeting minority communities. This translates directly to legal contexts, impacting sentencing and parole decisions.
- Contract analysis tools: AI systems designed to analyze contracts may misinterpret or undervalue agreements involving parties from marginalized groups due to biased training data.
- Legal research platforms: If the underlying data used by legal research AI is skewed, the results returned may not be truly representative or inclusive, potentially leading to unfair or inaccurate legal strategies.
Inaccuracy and the Limits of Legal AI
Beyond bias, inaccuracy remains a significant hurdle. Survey data indicates that many legal AI systems struggle with the nuances and complexities of legal language and reasoning. This can lead to several issues:
Sources of Inaccuracy:
- Data limitations: Insufficient or poorly curated training data can result in inaccurate predictions and flawed analyses.
- Ambiguity in legal language: Legal language is often complex and ambiguous, posing significant challenges for AI systems designed for precise interpretation.
- Lack of context awareness: AI may struggle to understand the broader context of a legal case, leading to misinterpretations and erroneous conclusions.
Survey Data Highlights: Key Findings
While specific survey data varies depending on the study, several recurring themes emerge:
- High rates of inaccuracy reported by legal professionals: Surveys frequently show that a substantial portion of legal professionals using AI tools have encountered instances of inaccurate outputs.
- Underrepresentation of certain demographics in training data: Many studies highlight the underrepresentation of minority groups in the data used to train legal AI, directly contributing to biased outcomes.
- Lack of transparency in AI algorithms: A lack of transparency regarding the inner workings of AI systems makes it difficult to identify and address biases and inaccuracies effectively.
Mitigating Bias and Improving Accuracy: Practical Steps
Addressing the challenges of bias and inaccuracy in legal AI requires a multi-pronged approach:
- Data diversification: Ensure training datasets are diverse and representative of the broader population, actively including data from underrepresented groups.
- Algorithm transparency: Develop more transparent AI algorithms that allow for scrutiny and identification of potential biases.
- Human oversight: Maintain robust human oversight in the use of legal AI, allowing human experts to review and validate AI-generated outputs.
- Continuous monitoring and evaluation: Regularly assess the performance of legal AI systems to detect and correct biases and inaccuracies.
- Investing in explainable AI (XAI): Prioritizing the development and use of explainable AI allows for better understanding of the reasoning behind AI's decisions, enhancing trust and accountability.
Conclusion: The Path Forward
The potential benefits of AI in the legal field are undeniable. However, addressing the issues of bias and inaccuracy is crucial to ensure fairness, equity, and the integrity of legal processes. By implementing the strategies outlined above, we can strive towards a future where legal AI serves as a valuable tool, enhancing rather than undermining justice. Let's focus on building more robust, ethical, and accurate AI systems for a more equitable legal landscape.
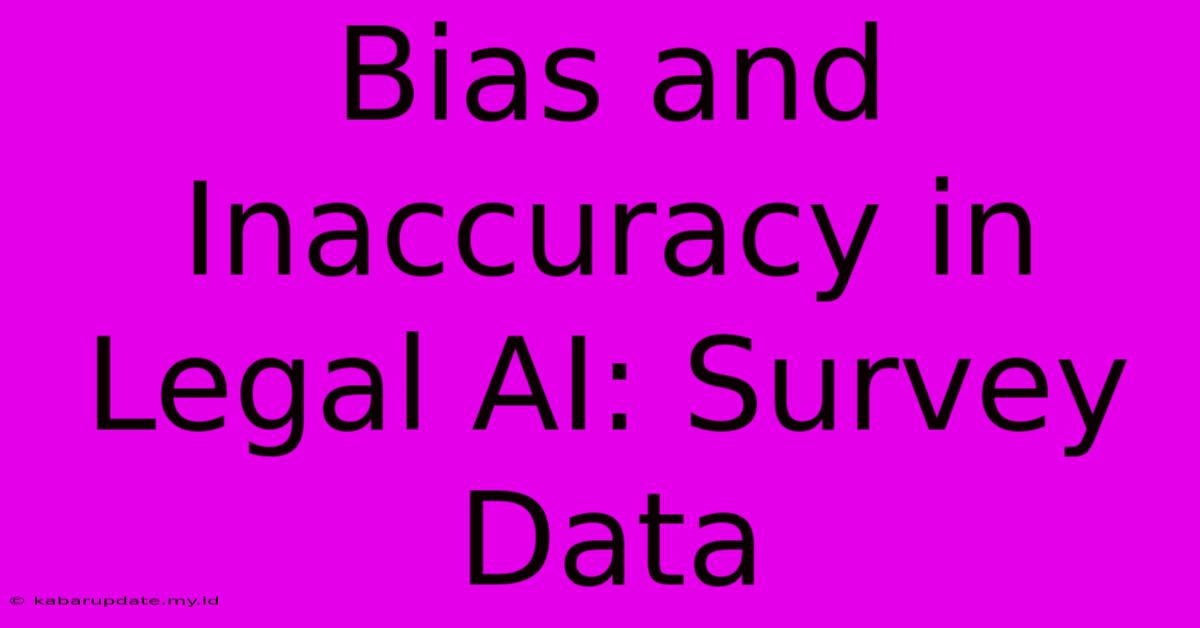
Thank you for taking the time to explore our website Bias And Inaccuracy In Legal AI: Survey Data. We hope you find the information useful. Feel free to contact us for any questions, and donβt forget to bookmark us for future visits!
We truly appreciate your visit to explore more about Bias And Inaccuracy In Legal AI: Survey Data. Let us know if you need further assistance. Be sure to bookmark this site and visit us again soon!
Featured Posts
-
Chennai Airport Turtle Seizure 5400 Red Eared Sliders
Dec 13, 2024
-
Proveryayte Chitaemost Teksta Ispolzuyte Prostye I Ponyatnye Predlozheniya Chtoby Maksimizirovat Chitaemost Vashego Kontenta
Dec 13, 2024
-
Resultados Empresariais Acores Impacto Sata
Dec 13, 2024
-
Harry O Rozwodzie Wspolczuje
Dec 13, 2024
-
Jude Law In Star Wars Succes
Dec 13, 2024