Algoritmos Y Sesgo Humano: Un Análisis
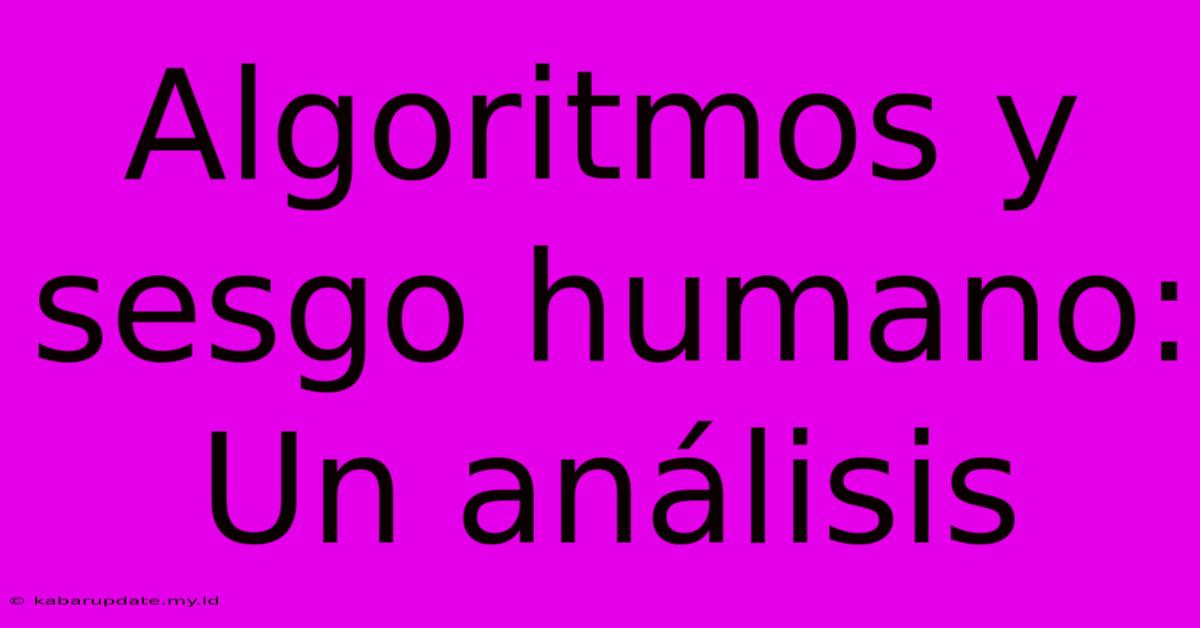
Discover more in-depth information on our site. Click the link below to dive deeper: Visit the Best Website meltwatermedia.ca. Make sure you don’t miss it!
Table of Contents
Algoritmos y Sesgo Humano: Un Análisis
The increasing reliance on algorithms in decision-making processes across various sectors raises crucial questions about their potential for bias. While algorithms are designed to be objective, they are, in fact, products of human creation and are susceptible to reflecting and even amplifying existing societal biases. This article explores the complex relationship between algorithms and human bias, examining its implications and offering strategies for mitigation.
The Inherent Bias in Algorithmic Design
Algorithms, at their core, are sets of rules and instructions. However, these rules are defined by humans, and human biases inevitably seep into the design and implementation phases. This can manifest in several ways:
1. Biased Data Sets:
The "garbage in, garbage out" principle applies strongly here. Algorithms are trained on data, and if that data reflects existing societal biases (e.g., gender, racial, or socioeconomic), the algorithm will learn and perpetuate those biases. For example, a facial recognition system trained primarily on images of white faces might perform poorly on individuals with darker skin tones.
2. Biased Feature Selection:
Even with unbiased data, the choice of features used to train an algorithm can introduce bias. If certain relevant factors are overlooked or weighted inappropriately, the algorithm's output can be skewed. Consider a loan application algorithm: if it prioritizes credit score over factors like employment history or education level, it might disproportionately disadvantage individuals from lower socioeconomic backgrounds.
3. Biased Interpretation of Results:
Finally, even with a well-designed algorithm, the interpretation of its results can introduce bias. Humans might selectively focus on aspects that confirm their pre-existing beliefs, ignoring contradictory evidence.
The Consequences of Algorithmic Bias
The implications of biased algorithms are far-reaching and can have serious consequences:
- Discrimination: Biased algorithms can perpetuate and even exacerbate discrimination in areas like hiring, loan applications, and criminal justice.
- Erosion of Trust: When algorithms consistently produce unfair or inaccurate results, it can lead to a loss of public trust in technology and institutions.
- Reinforcement of Social Inequalities: Biased algorithms can reinforce existing social inequalities by providing unfair advantages to certain groups while disadvantaging others.
Mitigating Algorithmic Bias
Addressing algorithmic bias requires a multi-pronged approach:
1. Data Diversity and Auditing:
Ensure the data used to train algorithms is diverse and representative of the population it will affect. Regular audits are crucial to identify and address biases in the data and the algorithm's performance.
2. Algorithmic Transparency and Explainability:
Increase transparency in how algorithms work. "Explainable AI" (XAI) techniques help make algorithmic decision-making more understandable, allowing for identification and correction of biases.
3. Human Oversight and Intervention:
While automation is efficient, human oversight remains essential. Human review can help catch biases that might otherwise go unnoticed by the algorithm.
4. Continuous Monitoring and Improvement:
Algorithms should not be considered static entities. Continuous monitoring of their performance and iterative improvements are necessary to adapt to changing circumstances and mitigate emerging biases.
Conclusion: Towards Fairer Algorithms
The problem of algorithmic bias is complex, requiring a concerted effort from developers, policymakers, and society as a whole. By actively addressing the sources of bias and implementing strategies for mitigation, we can work towards creating algorithms that are fair, equitable, and beneficial for all. Let's strive for a future where technology serves to enhance, not undermine, social justice.
Call to Action: What steps can your organization take to ensure fairness and equity in its algorithmic systems? Share your thoughts and experiences in the comments below!
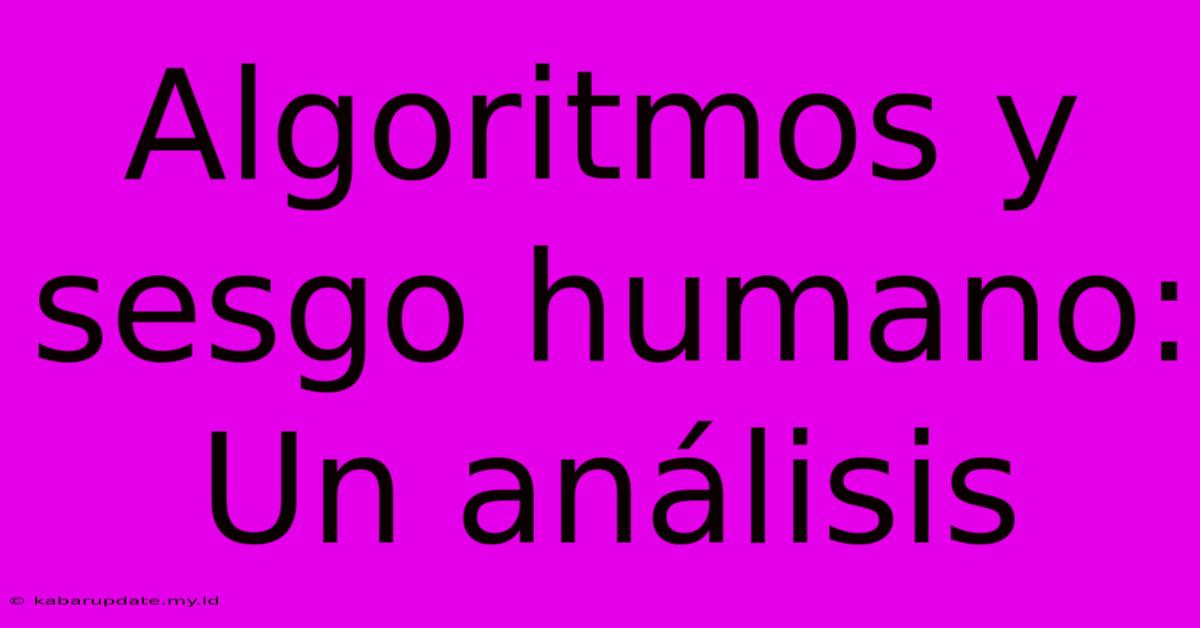
Thank you for taking the time to explore our website Algoritmos Y Sesgo Humano: Un Análisis. We hope you find the information useful. Feel free to contact us for any questions, and don’t forget to bookmark us for future visits!
We truly appreciate your visit to explore more about Algoritmos Y Sesgo Humano: Un Análisis. Let us know if you need further assistance. Be sure to bookmark this site and visit us again soon!
Featured Posts
-
Fengal Cyclone Unpredictable Track Analysis
Dec 11, 2024
-
Death Of United Healthcare Ceo Brian Thompson
Dec 11, 2024
-
United Healthcare Ceo Death New York Shooting
Dec 11, 2024
-
Karnataka Rains Several Areas Hit
Dec 11, 2024
-
La Dictadura Del Algoritmo Neutralidad O Sesgo
Dec 11, 2024