AI Adoption Gap: Solutions
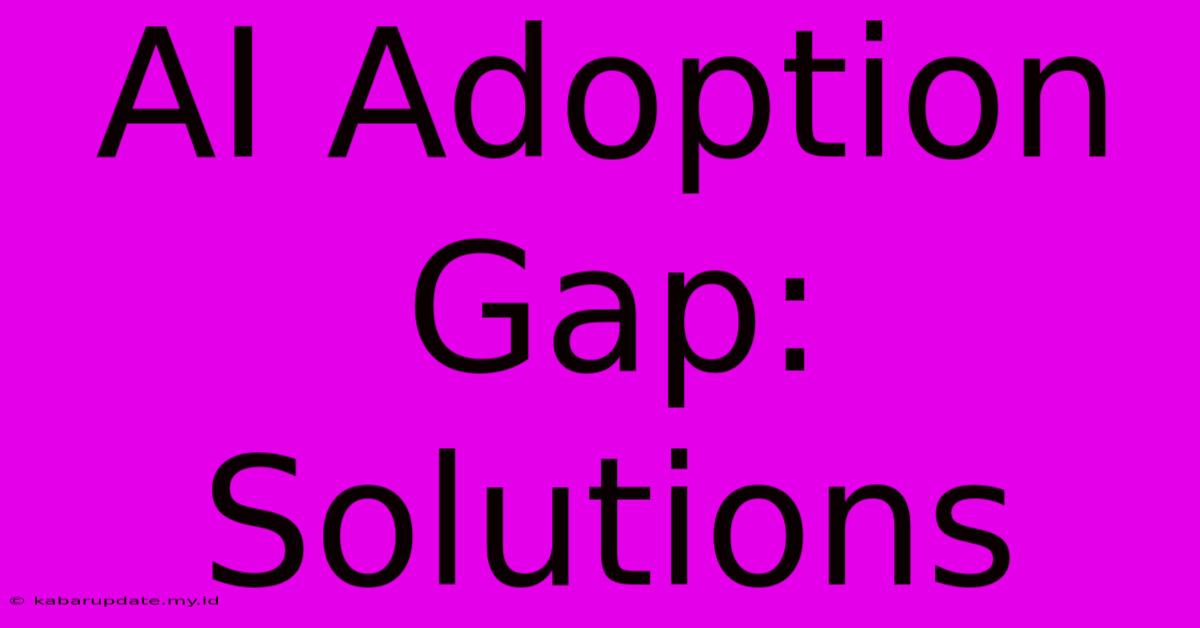
Discover more in-depth information on our site. Click the link below to dive deeper: Visit the Best Website meltwatermedia.ca. Make sure you donβt miss it!
Table of Contents
AI Adoption Gap: Bridging the Chasm Between Potential and Reality
Artificial intelligence (AI) promises a revolution across industries, from streamlining operations to fostering innovation. Yet, a significant gap exists between the potential of AI and its actual adoption. Many businesses understand the transformative power of AI, but struggle to implement it effectively. This article explores the key challenges hindering AI adoption and offers practical solutions to bridge this gap.
Understanding the AI Adoption Gap
The AI adoption gap isn't simply about a lack of technology; it's a multifaceted problem stemming from several interconnected factors:
1. Lack of Skilled Talent:
Finding and retaining data scientists, AI engineers, and machine learning specialists is a major hurdle. The demand far outweighs the supply, leading to high salaries and fierce competition for talent.
2. Data Challenges:
AI algorithms thrive on data. However, many organizations struggle with data quality, accessibility, and integration. Poor data quality leads to inaccurate models and unreliable results, hindering AI implementation.
3. High Implementation Costs:
Developing, deploying, and maintaining AI systems can be expensive. This includes the cost of hardware, software, talent, and ongoing maintenance. The significant upfront investment can be prohibitive for smaller businesses.
4. Integration Complexity:
Integrating AI solutions into existing infrastructure and workflows can be incredibly complex. Legacy systems often lack the necessary APIs and compatibility, requiring substantial modifications or replacements.
5. Lack of Clear ROI:
Demonstrating a clear return on investment (ROI) for AI initiatives is crucial for securing buy-in from stakeholders. The intangible benefits of AI can be difficult to quantify, making it challenging to justify the investment.
6. Security and Ethical Concerns:
Concerns about data security, algorithmic bias, and the ethical implications of AI are increasingly prevalent. Addressing these concerns is vital for building trust and ensuring responsible AI adoption.
Bridging the Gap: Practical Solutions
Overcoming the AI adoption gap requires a multi-pronged approach:
1. Invest in Talent Development:
Organizations should invest in training programs to upskill their existing workforce and attract new talent. Collaborations with universities and bootcamps can help build a pipeline of skilled AI professionals.
2. Improve Data Management:
Implementing robust data governance strategies, investing in data quality tools, and establishing clear data ownership are essential. Data cleaning, integration, and standardization are crucial for accurate AI models.
3. Start Small and Iterate:
Begin with pilot projects focused on specific business problems. This allows for a phased approach, minimizing risk and demonstrating early wins that justify further investment. Iterative development allows for continuous improvement based on real-world feedback.
4. Leverage Cloud-Based AI Solutions:
Cloud platforms offer readily available AI tools and services, reducing the need for significant upfront investment in infrastructure. This lowers the barrier to entry for smaller businesses and allows for scalable deployment.
5. Focus on Measurable Outcomes:
Define clear KPIs and metrics to track the success of AI initiatives. This allows for the quantification of benefits, demonstrating a clear ROI to stakeholders and justifying continued investment.
6. Prioritize Ethical Considerations:
Develop clear guidelines and ethical frameworks for AI development and deployment. Address potential biases in algorithms and ensure data privacy and security.
Conclusion
The AI adoption gap presents a significant challenge, but itβs not insurmountable. By addressing the key obstacles and implementing the solutions outlined above, organizations can harness the transformative power of AI, driving efficiency, innovation, and growth. Remember that a proactive and strategic approach, coupled with a commitment to continuous learning and adaptation, is key to successful AI implementation. Start small, focus on clear objectives, and prioritize ethical considerations β this is the pathway to bridging the gap and realizing the full potential of AI.
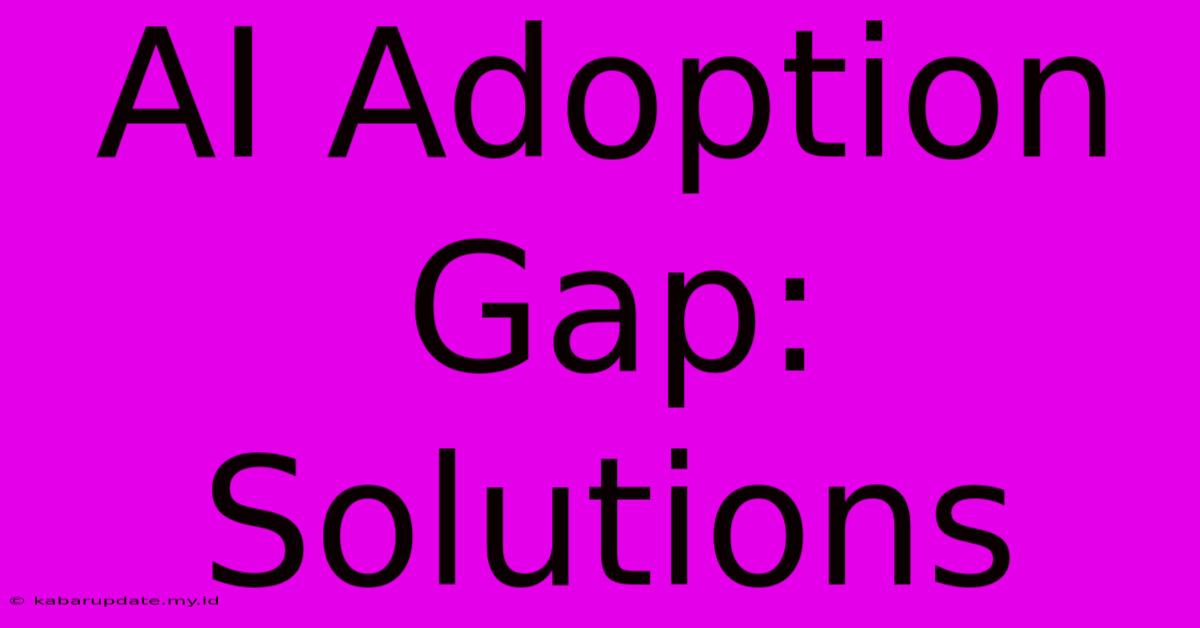
Thank you for taking the time to explore our website AI Adoption Gap: Solutions. We hope you find the information useful. Feel free to contact us for any questions, and donβt forget to bookmark us for future visits!
We truly appreciate your visit to explore more about AI Adoption Gap: Solutions. Let us know if you need further assistance. Be sure to bookmark this site and visit us again soon!
Featured Posts
-
Aktor Snape A Zaskakujace Doniesienia
Dec 13, 2024
-
Legal Ai Survey Bias And Inaccuracy Risks
Dec 13, 2024
-
Buenos Aires Trap Duki Bizarrap Nicki Nicole
Dec 13, 2024
-
Pikem Donk Team Spirit I Pley Off Meydzhora Etot Zagolovok Yavlyaetsya Korotkim Yomkim I Pryamo Ukazyvaet Na Temu On Khorosho Podkhodit Dlya Shirokoy Auditorii
Dec 13, 2024
-
Snape W Harrym Potterze Nowe Fakty
Dec 13, 2024