AI Adoption Challenges & Solutions
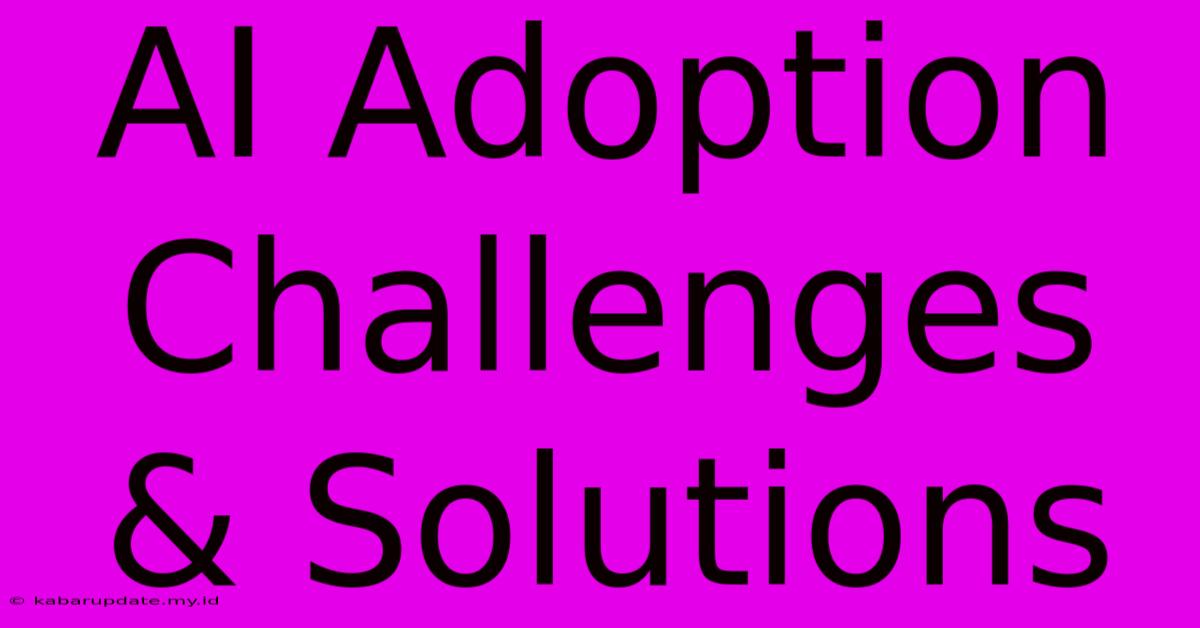
Discover more in-depth information on our site. Click the link below to dive deeper: Visit the Best Website meltwatermedia.ca. Make sure you donβt miss it!
Table of Contents
AI Adoption Challenges & Solutions: Overcoming Barriers to Intelligent Transformation
Artificial intelligence (AI) is rapidly transforming industries, offering unprecedented opportunities for automation, efficiency, and innovation. However, the journey to successful AI adoption is not without its hurdles. Many organizations face significant challenges in integrating AI into their operations. This article explores common obstacles and provides practical solutions to help businesses navigate the path to intelligent transformation.
Key Challenges in AI Adoption
Several factors hinder the widespread and effective adoption of AI technologies. These challenges span technical, organizational, and ethical considerations:
1. Data Challenges: The Fuel of AI
AI models, particularly machine learning algorithms, are data-hungry. Insufficient, low-quality, or inaccessible data severely limits AI performance.
- Challenge: Lack of sufficient, relevant, and high-quality data; data silos within organizations; difficulty in data cleaning and preprocessing; data security and privacy concerns.
- Solution: Invest in robust data management strategies. Implement data governance policies to ensure data quality and accessibility. Utilize data integration tools to consolidate data from various sources. Prioritize data privacy and security measures, complying with regulations like GDPR. Explore data augmentation techniques to expand limited datasets.
2. Lack of Skilled Talent: The Human Element
Developing, deploying, and maintaining AI systems requires specialized expertise. A shortage of skilled data scientists, AI engineers, and machine learning specialists is a major bottleneck.
- Challenge: Difficulty in recruiting and retaining AI talent; lack of internal expertise to manage AI projects; high cost of hiring external consultants.
- Solution: Invest in employee training and upskilling programs to build internal AI expertise. Partner with universities and educational institutions to access talent pipelines. Consider outsourcing specific AI tasks to specialized firms. Leverage low-code/no-code AI platforms to empower citizen data scientists.
3. Integration Complexity: Connecting the Dots
Integrating AI systems into existing IT infrastructure and business processes can be complex and time-consuming. Compatibility issues and legacy systems often pose significant obstacles.
- Challenge: Difficulty in integrating AI systems with existing software and hardware; lack of standardized APIs; challenges in scaling AI solutions; high integration costs.
- Solution: Choose AI solutions designed for seamless integration with your existing infrastructure. Employ a phased approach to AI implementation, starting with pilot projects. Invest in robust API management and integration platforms. Prioritize cloud-based AI solutions for scalability and flexibility.
4. Cost and ROI: Justifying the Investment
Implementing and maintaining AI systems can be expensive, involving significant upfront investment in hardware, software, talent, and training. Demonstrating a clear return on investment (ROI) is crucial.
- Challenge: High initial investment costs; difficulty in quantifying the ROI of AI projects; lack of clear business case for AI adoption.
- Solution: Conduct thorough cost-benefit analyses to justify AI investments. Start with small-scale pilot projects to demonstrate ROI before scaling up. Focus on AI solutions that address specific business problems with measurable outcomes.
5. Ethical Considerations: Responsible AI
Ethical concerns surrounding AI, such as bias in algorithms, job displacement, and data privacy, are increasingly important. Addressing these concerns is critical for building trust and ensuring responsible AI adoption.
- Challenge: Potential for algorithmic bias; concerns about job displacement; data privacy violations; lack of ethical guidelines for AI development and deployment.
- Solution: Implement rigorous testing and monitoring procedures to detect and mitigate bias in AI models. Develop transparent and explainable AI systems. Prioritize data privacy and security. Establish clear ethical guidelines for AI development and deployment, ensuring compliance with relevant regulations.
Successfully Navigating the Challenges: Practical Tips
- Start Small, Think Big: Begin with pilot projects focused on specific business problems. This allows you to gain experience, demonstrate ROI, and refine your approach before scaling up.
- Foster Collaboration: Encourage collaboration between IT, business, and data science teams. This ensures alignment between AI initiatives and business goals.
- Invest in Education and Training: Develop internal AI expertise through training and upskilling programs.
- Choose the Right Tools: Select AI solutions tailored to your specific needs and existing infrastructure.
- Monitor and Evaluate: Regularly monitor the performance of your AI systems and evaluate their impact on your business.
By addressing these challenges proactively and implementing the solutions outlined above, organizations can unlock the transformative potential of AI and achieve a successful intelligent transformation. Remember, the journey to AI adoption is an iterative process requiring continuous learning, adaptation, and a commitment to responsible AI practices.
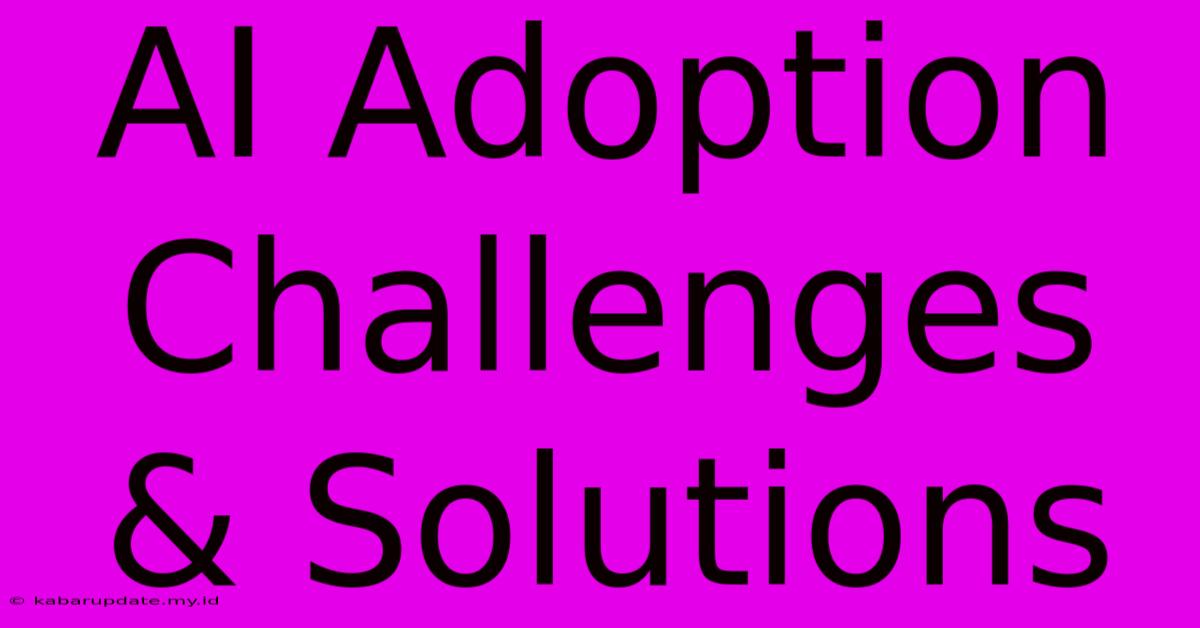
Thank you for taking the time to explore our website AI Adoption Challenges & Solutions. We hope you find the information useful. Feel free to contact us for any questions, and donβt forget to bookmark us for future visits!
We truly appreciate your visit to explore more about AI Adoption Challenges & Solutions. Let us know if you need further assistance. Be sure to bookmark this site and visit us again soon!
Featured Posts
-
Red Eared Slider Seizure Chennai Airport Bust
Dec 13, 2024
-
Exploring Female Ai Adoption Rates
Dec 13, 2024
-
Wywiad Harry Ego Porazka
Dec 13, 2024
-
Declaraciones Ramos Rizo Penal Ocampos
Dec 13, 2024
-
Recessao E Falta De Mao De Obra Preocupam Lideres
Dec 13, 2024