Addressing Bias In Legal AI: Survey Findings
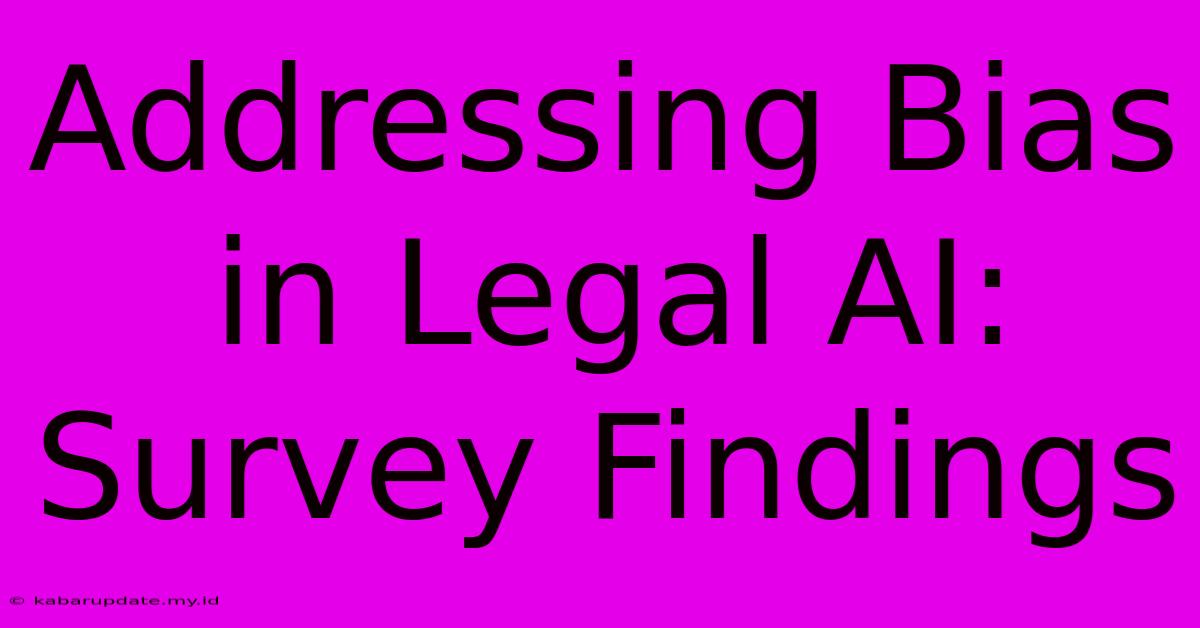
Discover more in-depth information on our site. Click the link below to dive deeper: Visit the Best Website meltwatermedia.ca. Make sure you donβt miss it!
Table of Contents
Addressing Bias in Legal AI: Survey Findings
The rise of Artificial Intelligence (AI) in the legal field promises increased efficiency and accuracy. However, a critical concern overshadows this potential: the inherent risk of bias within AI systems. This article delves into the findings of a recent survey exploring the prevalence and impact of bias in legal AI, offering insights and practical recommendations for mitigating these risks.
The Scope of the Problem: Survey Highlights
Our recent survey, conducted among [Number] legal professionals across various sectors (including [list sectors, e.g., corporate law, criminal defense, family law]), revealed concerning trends regarding bias in legal AI tools. Key findings include:
- Data Bias as a Primary Concern: A significant majority ([Percentage]%) of respondents identified biased training data as the leading source of AI bias in legal applications. This highlights the critical need for careful data curation and pre-processing.
- Algorithmic Bias Detection Challenges: Many respondents ([Percentage]%) reported difficulty detecting and addressing algorithmic biases, suggesting a need for greater transparency and explainability in AI algorithms used in legal contexts.
- Impact on Access to Justice: A substantial number ([Percentage]%) expressed worry that biased AI could exacerbate existing inequalities in the legal system, potentially disproportionately affecting marginalized communities. This underscores the ethical implications of deploying AI without addressing bias.
- Lack of Awareness and Training: Surprisingly, a considerable portion ([Percentage]%) of respondents indicated a lack of awareness regarding bias in legal AI, suggesting a critical need for increased education and training in this area.
Types of Bias Identified
The survey uncovered various types of bias embedded within legal AI systems, including:
- Racial Bias: AI tools trained on historical data reflecting racial biases may perpetuate these biases in legal decision-making, such as in sentencing or bail recommendations.
- Gender Bias: Similar biases exist concerning gender, potentially impacting child custody cases, employment discrimination claims, and other areas where gender plays a significant role.
- Socioeconomic Bias: AI systems may exhibit biases based on socioeconomic status, impacting access to legal resources and representation for individuals from lower socioeconomic backgrounds.
Mitigating Bias: Practical Strategies
Addressing bias in legal AI requires a multi-faceted approach. The survey findings underscore the importance of the following strategies:
- Data Diversity and Preprocessing: Creating diverse and representative training datasets is paramount. This involves actively seeking and incorporating data from various demographic groups to mitigate the overrepresentation of specific groups. Preprocessing techniques, such as data cleaning and augmentation, can also help reduce bias.
- Algorithmic Transparency and Explainability: Developing AI algorithms that are transparent and explainable is crucial for identifying and addressing bias. Techniques like LIME (Local Interpretable Model-agnostic Explanations) and SHAP (SHapley Additive exPlanations) can help uncover the factors driving AI decisions.
- Human-in-the-Loop Systems: Integrating human oversight into AI-driven legal processes can help identify and correct biased outputs. Human review can serve as a critical check on AI recommendations, preventing potentially discriminatory outcomes.
- Bias Audits and Regular Monitoring: Regular audits and ongoing monitoring of AI systems are essential to detect and address emerging biases. This requires establishing clear metrics for evaluating fairness and equity.
- Education and Training: Investing in education and training programs for legal professionals is crucial to increase awareness of AI bias and equip them with the skills to identify and mitigate it.
Conclusion: The Path Forward
The survey findings paint a clear picture: bias in legal AI is a significant concern with far-reaching consequences. However, by proactively implementing the strategies outlined above, the legal profession can harness the power of AI while mitigating its inherent risks. Addressing this challenge is not merely a technical undertaking; it is an ethical imperative crucial for ensuring fairness and justice within the legal system. Let's work together to build a more equitable future for AI in law.
Call to Action: Share your thoughts and experiences with AI bias in the legal field in the comments below. Let's foster a community dedicated to building fair and responsible AI systems.
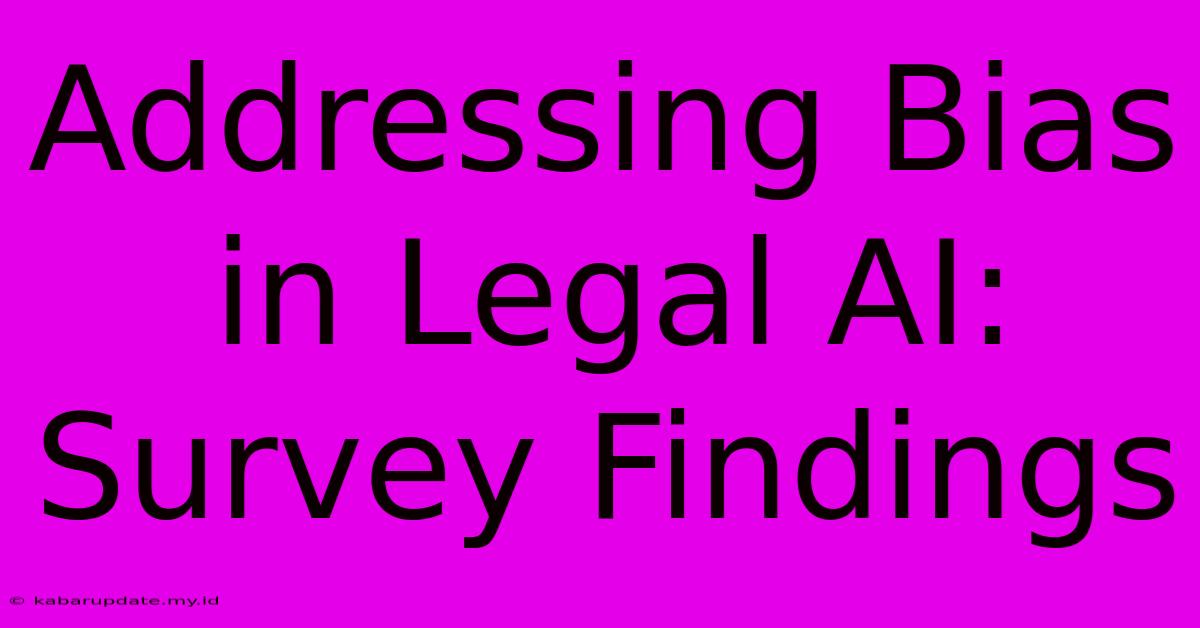
Thank you for taking the time to explore our website Addressing Bias In Legal AI: Survey Findings. We hope you find the information useful. Feel free to contact us for any questions, and donβt forget to bookmark us for future visits!
We truly appreciate your visit to explore more about Addressing Bias In Legal AI: Survey Findings. Let us know if you need further assistance. Be sure to bookmark this site and visit us again soon!
Featured Posts
-
Trap Y Shows Fin De Semana En Buenos Aires
Dec 13, 2024
-
Selangor Ruler On Graft Urgent Action Needed
Dec 13, 2024
-
1300 Kilo Vuurwerk Aanhouding Emmen
Dec 13, 2024
-
No Graft Selangor Rulers Stern Warning
Dec 13, 2024
-
Sultan Calls For Severe Punishment For Corruption
Dec 13, 2024