Accuracy Concerns In Legal AI Systems: Survey
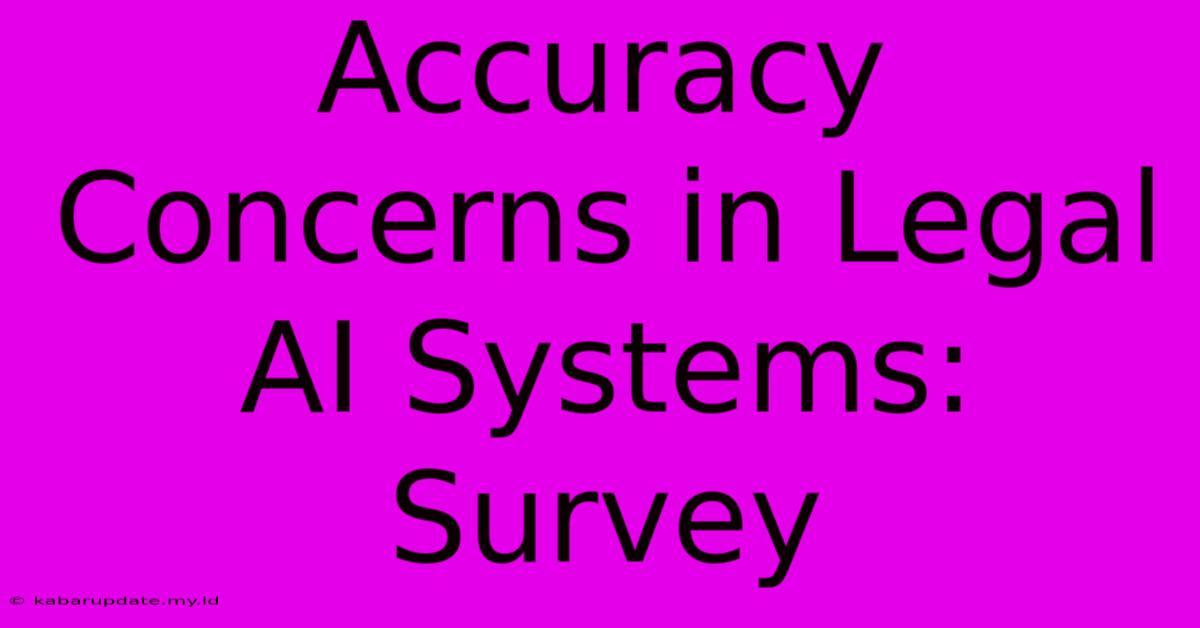
Discover more in-depth information on our site. Click the link below to dive deeper: Visit the Best Website meltwatermedia.ca. Make sure you donβt miss it!
Table of Contents
Accuracy Concerns in Legal AI Systems: A Survey
The rise of Artificial Intelligence (AI) is transforming numerous industries, and the legal field is no exception. AI-powered tools promise increased efficiency and accuracy in tasks ranging from contract review to legal research. However, concerns about the accuracy of these systems are growing, prompting a need for careful examination and robust mitigation strategies. This article surveys the key accuracy concerns surrounding legal AI and offers practical advice for mitigating risks.
The Promise and Peril of Legal AI
Legal AI systems offer the potential to revolutionize legal practice. They can process vast amounts of data quickly, identify relevant precedents, and predict case outcomes with greater speed than human lawyers. This efficiency can translate into cost savings and improved client service. However, the accuracy of these predictions and analyses depends heavily on the quality of the data used to train the AI models and the inherent limitations of the algorithms themselves.
Data Bias: A Major Source of Inaccuracy
One primary concern is data bias. AI models are trained on existing datasets, and if these datasets reflect existing biases within the legal system (e.g., racial, gender, or socioeconomic biases in sentencing or case outcomes), the AI will likely perpetuate and even amplify these biases. This can lead to inaccurate and unfair outcomes. For example, an AI trained on historical data showing disproportionate sentencing for certain demographics might predict harsher sentences for individuals from those same demographics, even if their individual circumstances warrant a lighter sentence.
Limited Understanding of Nuance and Context
Legal reasoning often involves subtle nuances, context-dependent interpretations, and ethical considerations that are challenging for AI to grasp. Current AI models excel at pattern recognition but struggle with the kind of nuanced understanding and judgment required for complex legal decisions. A contract review AI, for instance, might miss crucial clauses or misinterpret ambiguous language, leading to inaccurate assessments of risk or liability.
Lack of Transparency and Explainability
Many AI systems, particularly deep learning models, operate as "black boxes," making it difficult to understand how they arrive at their conclusions. This lack of transparency makes it challenging to identify and correct errors, hindering trust and accountability. If an AI system makes a critical error in a legal case, understanding why the error occurred is crucial for rectifying the situation and preventing future mistakes. The inability to explain AI decisions poses significant challenges for legal professionals who need to justify their actions and decisions in court.
Mitigating Accuracy Concerns in Legal AI
Despite the challenges, several strategies can help mitigate accuracy concerns in legal AI:
- Data Cleansing and Bias Mitigation: Before training an AI model, it's crucial to thoroughly cleanse the data to remove biases and inconsistencies. Techniques like data augmentation and re-weighting can help balance datasets and reduce the impact of existing biases.
- Human-in-the-Loop Systems: Integrating human oversight into the AI workflow is critical. Legal professionals should review and validate the AI's outputs, ensuring accuracy and correcting any errors or biases. This collaborative approach leverages the strengths of both humans and AI.
- Explainable AI (XAI): Developing and implementing XAI techniques is vital. XAI aims to make AI decision-making more transparent and understandable, facilitating error detection and improving trust.
- Continuous Monitoring and Evaluation: Regularly monitoring the AI system's performance and evaluating its accuracy is crucial for identifying and addressing emerging problems. This involves tracking metrics such as precision, recall, and F1-score, and comparing AI outputs to human expert judgments.
- Focus on Specific Tasks: Instead of attempting to create a single, all-encompassing AI system for all legal tasks, focusing on developing AI for specific, well-defined tasks can yield more accurate and reliable results.
Conclusion
While accuracy concerns remain a significant hurdle for widespread adoption of legal AI, proactive measures like those described above can pave the way for responsible and reliable AI implementation in the legal sector. The key lies in a collaborative approach that combines the strengths of human expertise and AI capabilities while prioritizing transparency, accountability, and fairness. By addressing these concerns, the legal profession can harness the power of AI to improve efficiency, access to justice, and overall accuracy in legal practice.
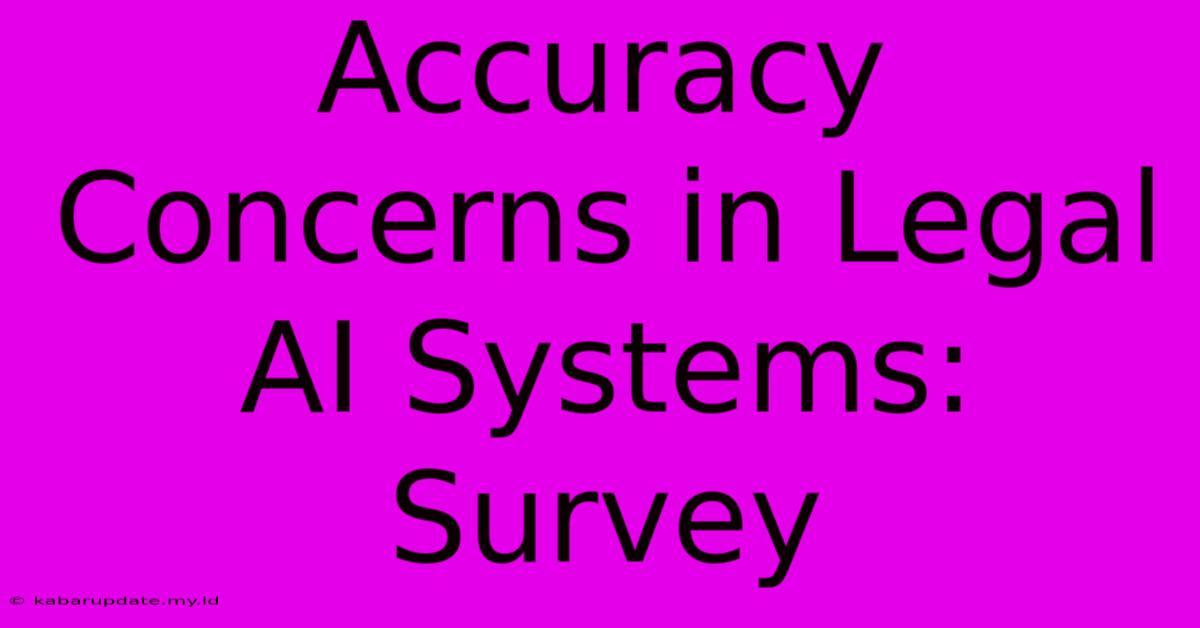
Thank you for taking the time to explore our website Accuracy Concerns In Legal AI Systems: Survey. We hope you find the information useful. Feel free to contact us for any questions, and donβt forget to bookmark us for future visits!
We truly appreciate your visit to explore more about Accuracy Concerns In Legal AI Systems: Survey. Let us know if you need further assistance. Be sure to bookmark this site and visit us again soon!
Featured Posts
-
Harry Potter Obsada Snape A Rewelacje
Dec 13, 2024
-
Sultan Pushes For Special Court On Corruption Cases
Dec 13, 2024
-
Droga Serwisowa Ogrodzenie Elektryczne
Dec 13, 2024
-
Ravshan Defeats Wakrah In Gulf Times Match
Dec 13, 2024
-
Iccs Failures A Critical Analysis
Dec 13, 2024